2024
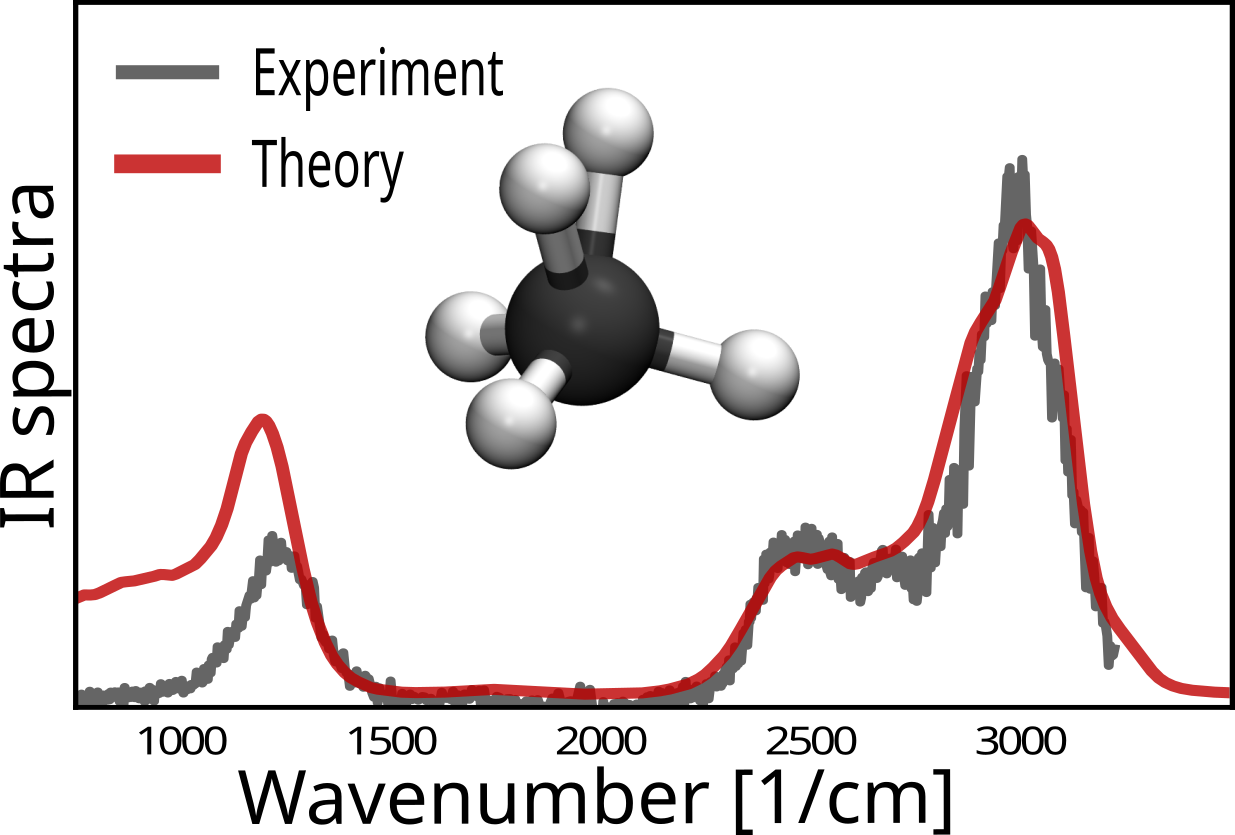
Richard Beckmann, Christoph Schran, Fabien Brieuc, Dominik Marx
Theoretical infrared spectroscopy of protonated methane isotopologues Journal Article
In: Phys. Chem. Chem. Phys., vol. 26, iss. 35, pp. 22846-22852, 2024.
Abstract | Links | BibTeX | Tags: Coupled Cluster, Quantum Dynamics, Spectra
@article{Beckmann2024/10.1039/D4CP02295E,
title = {Theoretical infrared spectroscopy of protonated methane isotopologues},
author = {Richard Beckmann and Christoph Schran and Fabien Brieuc and Dominik Marx},
url = {http://dx.doi.org/10.1039/D4CP02295E},
doi = {10.1039/D4CP02295E},
year = {2024},
date = {2024-08-13},
urldate = {2024-08-13},
journal = {Phys. Chem. Chem. Phys.},
volume = {26},
issue = {35},
pages = {22846-22852},
publisher = {The Royal Society of Chemistry},
abstract = {The vibrational spectroscopy of protonated methane and its mixed hydrogen/deuterium isotopologues remains a challenge to both experimental and computational spectroscopy due to the iconic floppiness of CH5+. Here, we compute the finite-temperature broadband infrared spectra of CH5+ and all its isotopologues, i.e. CHnD5−n+ up to CD5+, from path integral molecular dynamics in conjunction with interactions and dipoles computed consistently at CCSD(T) coupled cluster accuracy. The potential energy and dipole moment surfaces have been accurately represented in full dimensionality in terms of high-dimensional neural networks. The resulting computational efficiency allows us to establish CCSD(T) accuracy at the level of converged path integral simulations. For all six isotopologues, the computed broadband spectra compare very favorably to the available experimental broadband spectra obtained from laser induced reactions action vibrational spectroscopy. The current approach is found to consistently and significantly improve on previous calculations of these broadband vibrational spectra and defines the new cutting-edge for what has been dubbed the “enfant terrible” of molecular spectroscopy in view of its pronounced large-amplitude motion that involves all intramolecular degrees of freedom.},
keywords = {Coupled Cluster, Quantum Dynamics, Spectra},
pubstate = {published},
tppubtype = {article}
}
2022
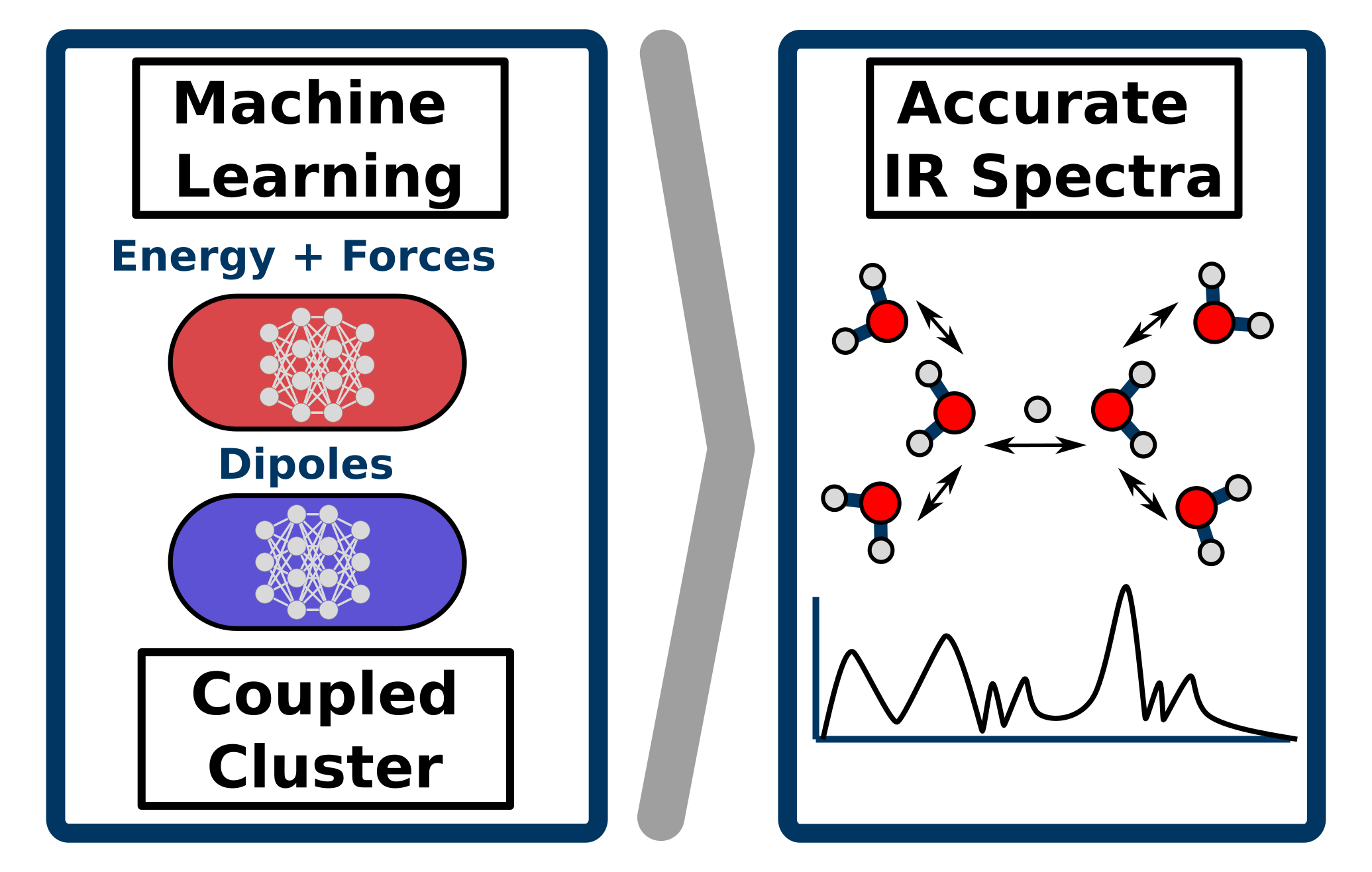
Richard Beckmann, Fabien Brieuc, Christoph Schran, Dominik Marx
Infrared spectra at coupled cluster accuracy from neural network representations Journal Article
In: J. Chem. Theory Comput., 2022, ISSN: 1549-9618.
Abstract | Links | BibTeX | Tags: Coupled Cluster, Machine Learning Potentials, Spectra, Water
@article{Beckmann2022/10.1021/ACS.JCTC.2C00511,
title = {Infrared spectra at coupled cluster accuracy from neural network representations},
author = {Richard Beckmann and Fabien Brieuc and Christoph Schran and Dominik Marx},
doi = {10.1021/ACS.JCTC.2C00511},
issn = {1549-9618},
year = {2022},
date = {2022-08-01},
urldate = {2022-08-01},
journal = {J. Chem. Theory Comput.},
publisher = {American Chemical Society},
abstract = {Infrared spectroscopy is key to elucidate molecular structures, monitor reactions and observe conformational changes, while providing information on both structural and dynamical properties. This makes the accurate prediction of infrared spectra based on first-principle theories a highly desirable pursuit. Molecular dynamics simulations have proven to be a particularly powerful approach for this task, albeit requiring the computation of energies, forces and dipole moments for a large number of molecular configurations as a function of time. This explains why highly accurate first principles methods, such as coupled cluster theory, have so far been inapplicable for the prediction of fully anharmonic vibrational spectra of large systems at finite temperatures. Here, we push cutting-edge machine learning techniques forward by using neural network representations of energies, forces and in particular dipoles to predict such infrared spectra fully at "gold standard" coupled cluster accuracy as demonstrated for protonated water clusters as large as the protonated water hexamer, in its extended Zundel configuration. Furthermore, we show that this methodology can be used beyond the scope of the data considered during the development of the neural network models, allowing for the computation of finite-temperature infrared spectra of large systems inaccessible to explicit coupled cluster calculations. This substantially expands the hitherto existing limits of accuracy, speed and system size for theoretical spectroscopy and opens up a multitude of avenues for the prediction of vibrational spectra and the understanding of complex intra- and intermolecular couplings.},
keywords = {Coupled Cluster, Machine Learning Potentials, Spectra, Water},
pubstate = {published},
tppubtype = {article}
}
2021
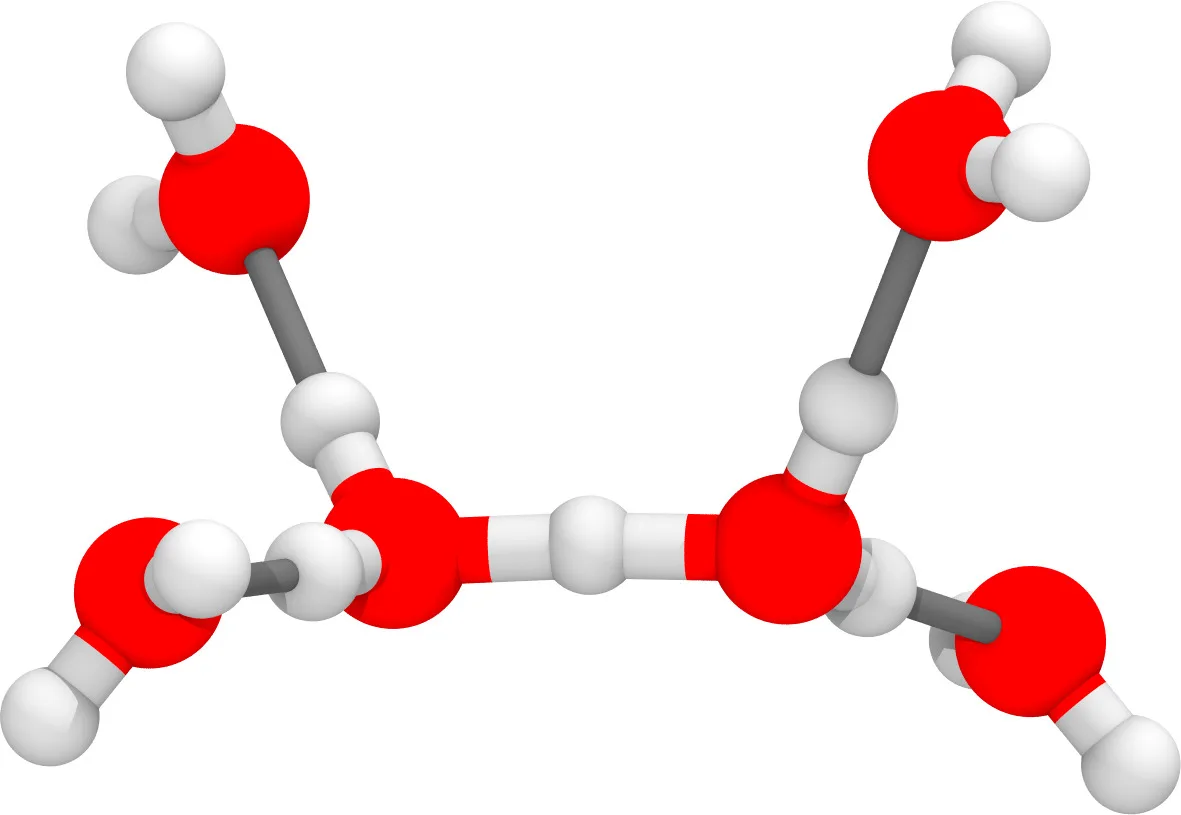
Christoph Schran, Fabien Brieuc, Dominik Marx
Transferability of machine learning potentials: Protonated water neural network potential applied to the protonated water hexamer Journal Article
In: J. Chem. Phys., vol. 154, no. 5, pp. 051101, 2021, ISSN: 10897690.
Abstract | Links | BibTeX | Tags: Coupled Cluster, Hydrogen bonding, Machine Learning Potentials, Water
@article{Schran2021/10.1063/5.0035438,
title = {Transferability of machine learning potentials: Protonated water neural network potential applied to the protonated water hexamer},
author = {Christoph Schran and Fabien Brieuc and Dominik Marx},
doi = {10.1063/5.0035438},
issn = {10897690},
year = {2021},
date = {2021-02-01},
urldate = {2021-02-01},
journal = {J. Chem. Phys.},
volume = {154},
number = {5},
pages = {051101},
abstract = {A previously published neural network potential for the description of protonated water clusters up to the protonated water tetramer, H+(H2O)4, at an essentially converged coupled cluster accuracy [C. Schran, J. Behler, and D. Marx, J. Chem. Theory Comput. 16, 88 (2020)] is applied to the protonated water hexamer, H+(H2O)6 - a system that the neural network has never seen before. Although being in the extrapolation regime, it is shown that the potential not only allows for quantum simulations from ultra-low temperatures ∼1 K up to 300 K but is also able to describe the new system very accurately compared to explicit coupled cluster calculations. This transferability of the model is rationalized by the similarity of the atomic environments encountered for the larger cluster compared to the environments in the training set of the model. Compared to the interpolation regime, the quality of the model is reduced by roughly one order of magnitude, but most of the difference to the coupled cluster reference comes from global shifts of the potential energy surface, while local energy fluctuations are well recovered. These results suggest that the application of neural network potentials in extrapolation regimes can provide useful results and might be more general than usually thought.},
keywords = {Coupled Cluster, Hydrogen bonding, Machine Learning Potentials, Water},
pubstate = {published},
tppubtype = {article}
}
2020
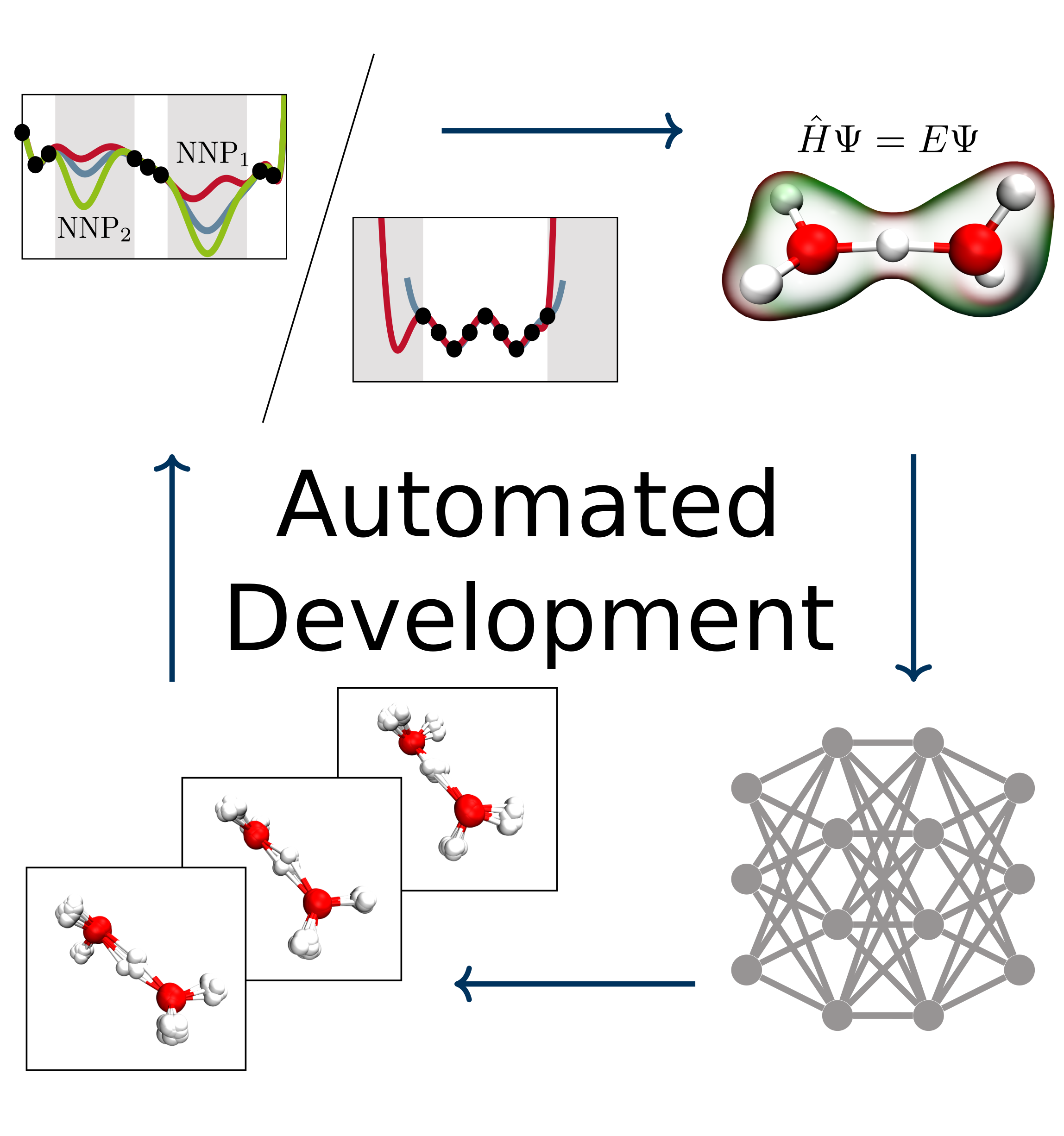
Christoph Schran, Jörg Behler, Dominik Marx
Automated Fitting of Neural Network Potentials at Coupled Cluster Accuracy: Protonated Water Clusters as Testing Ground Journal Article
In: J. Chem. Theory Comput., vol. 16, no. 1, pp. 88–99, 2020, ISSN: 15499626.
Abstract | Links | BibTeX | Tags: Coupled Cluster, Hydrogen bonding, Machine Learning Potentials, Water
@article{Schran2020/10.1021/acs.jctc.9b00805,
title = {Automated Fitting of Neural Network Potentials at Coupled Cluster Accuracy: Protonated Water Clusters as Testing Ground},
author = {Christoph Schran and Jörg Behler and Dominik Marx},
doi = {10.1021/acs.jctc.9b00805},
issn = {15499626},
year = {2020},
date = {2020-01-01},
urldate = {2020-01-01},
journal = {J. Chem. Theory Comput.},
volume = {16},
number = {1},
pages = {88–99},
abstract = {Highly accurate potential energy surfaces are of key interest for the detailed understanding and predictive modeling of chemical systems. In recent years, several new types of force fields, which are based on machine learning algorithms and fitted to ab initio reference calculations, have been introduced to meet this requirement. Here, we show how high-dimensional neural network potentials can be employed to automatically generate the potential energy surface of finite sized clusters at coupled cluster accuracy, namely CCSD(T*)-F12a/aug-cc-pVTZ. The developed automated procedure utilizes the established intrinsic properties of the model such that the configurations for the training set are selected in an unbiased and efficient way to minimize the computational effort of expensive reference calculations. These ideas are applied to protonated water clusters from the hydronium cation, H3O+, up to the tetramer, H9O4+, and lead to a single potential energy surface that describes all these systems at essentially converged coupled cluster accuracy with a fitting error of 0.06 kJ/mol per atom. The fit is validated in detail for all clusters up to the tetramer and yields reliable results not only for stationary points but also for reaction pathways and intermediate configurations as well as different sampling techniques. Per design, the neural network potentials (NNPs) constructed in this fashion can handle very different conditions including the quantum nature of the nuclei and enhanced sampling techniques covering very low as well as high temperatures. This enables fast and exhaustive exploration of the targeted protonated water clusters with essentially converged interactions. In addition, the automated process will allow one to tackle finite systems much beyond the present case.},
keywords = {Coupled Cluster, Hydrogen bonding, Machine Learning Potentials, Water},
pubstate = {published},
tppubtype = {article}
}