2024
Samuel G. H. Brookes, Venkat Kapil, Christoph Schran, Angelos Michaelides
The wetting of H$_2$O by CO$_2$ Journal Article
In: J. Chem. Phys., vol. 161, no. 8, pp. 084711, 2024, ISSN: 10897690.
Abstract | Links | BibTeX | Tags: Machine Learning Potentials, Water, Water at Interfaces
@article{Brookes2024/10.1063/5.0224230,
title = {The wetting of H$_2$O by CO$_2$},
author = {Samuel G. H. Brookes and Venkat Kapil and Christoph Schran and Angelos Michaelides},
url = {/aip/jcp/article/161/8/084711/3309975/The-wetting-of-H2O-by-CO2},
doi = {10.1063/5.0224230},
issn = {10897690},
year = {2024},
date = {2024-08-28},
urldate = {2024-08-28},
journal = {J. Chem. Phys.},
volume = {161},
number = {8},
pages = {084711},
publisher = {American Institute of Physics},
abstract = {Biphasic interfaces are complex but fascinating regimes that display a number of properties distinct from those of the bulk. The CO2-H2O interface, in particular, has been the subject of a number of studies on account of its importance for the carbon life cycle as well as carbon capture and sequestration schemes. Despite this attention, there remain a number of open questions on the nature of the CO2-H2O interface, particularly concerning the interfacial tension and phase behavior of CO2 at the interface. In this paper, we seek to address these ambiguities using ab initio-quality simulations. Harnessing the benefits of machine-learned potentials and enhanced statistical sampling methods, we present an ab initio-level description of the CO2-H2O interface. Interfacial tensions are predicted from 1 to 500 bars and found to be in close agreement with experiment at pressures for which experimental data are available. Structural analyses indicate the buildup of an adsorbed, saturated CO2 film forming at a low pressure (20 bars) with properties similar to those of the bulk liquid, but preferential perpendicular alignment with respect to the interface. The CO2 monolayer buildup coincides with a reduced structuring of water molecules close to the interface. This study highlights the predictive nature of machine-learned potentials for complex macroscopic properties of biphasic interfaces, and the mechanistic insight obtained into carbon dioxide aggregation at the water interface is of high relevance for geoscience, climate research, and materials science.},
keywords = {Machine Learning Potentials, Water, Water at Interfaces},
pubstate = {published},
tppubtype = {article}
}
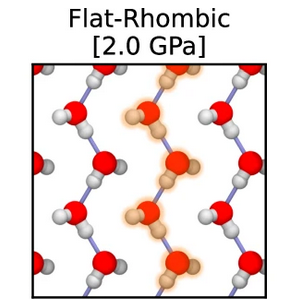
Pavan Ravindra, Xavier R. Advincula, Christoph Schran, Angelos Michaelides, Venkat Kapil
Quasi-one-dimensional hydrogen bonding in nanoconfined ice Journal Article
In: Nat. Commun., vol. 15, no. 1, pp. 1–9, 2024, ISSN: 20411723.
Abstract | Links | BibTeX | Tags: Confinement, Hydrogen bonding, Machine Learning Potentials, Water
@article{Ravindra2024/10.1038/s41467-024-51124-z,
title = {Quasi-one-dimensional hydrogen bonding in nanoconfined ice},
author = {Pavan Ravindra and Xavier R. Advincula and Christoph Schran and Angelos Michaelides and Venkat Kapil},
url = {https://www.nature.com/articles/s41467-024-51124-z},
doi = {10.1038/s41467-024-51124-z},
issn = {20411723},
year = {2024},
date = {2024-08-24},
urldate = {2024-08-24},
journal = {Nat. Commun.},
volume = {15},
number = {1},
pages = {1–9},
publisher = {Nature Publishing Group},
abstract = {The Bernal-Fowler ice rules stipulate that each water molecule in an ice crystal should form four hydrogen bonds. However, in extreme or constrained conditions, the arrangement of water molecules deviates from conventional ice rules, resulting in properties significantly different from bulk water. In this study, we employ machine learning-driven first-principles simulations to identify a new stabilization mechanism in nanoconfined ice phases. Instead of forming four hydrogen bonds, nanoconfined crystalline ice can form a quasi-one-dimensional hydrogen-bonded structure that exhibits only two hydrogen bonds per water molecule. These structures consist of strongly hydrogen-bonded linear chains of water molecules that zig-zag along one dimension, stabilized by van der Waals interactions that stack these chains along the other dimension. The unusual interplay of hydrogen bonding and van der Waals interactions in nanoconfined ice results in atypical proton behavior such as potential ferroelectric behavior, low dielectric response, and long-range proton dynamics.},
keywords = {Confinement, Hydrogen bonding, Machine Learning Potentials, Water},
pubstate = {published},
tppubtype = {article}
}
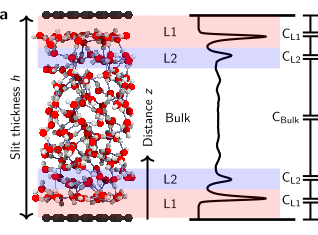
Thomas Dufils, Christoph Schran, Ji Chen, Andre K. Geim, Laura Fumagalli, Angelos Michaelides
Origin of dielectric polarization suppression in confined water from first principles Journal Article
In: Chem. Sci., vol. 15, iss. 2, pp. 516–527, 2024.
Abstract | Links | BibTeX | Tags: AIMD, Confinement, Hydrogen bonding, Water
@article{Dufils2024/10.1039/D3SC04740G,
title = {Origin of dielectric polarization suppression in confined water from first principles},
author = {Thomas Dufils and Christoph Schran and Ji Chen and Andre K. Geim and Laura Fumagalli and Angelos Michaelides},
url = {http://dx.doi.org/10.1039/D3SC04740G},
doi = {10.1039/D3SC04740G},
year = {2024},
date = {2024-01-01},
urldate = {2024-01-01},
journal = {Chem. Sci.},
volume = {15},
issue = {2},
pages = {516–527},
publisher = {The Royal Society of Chemistry},
abstract = {It has long been known that the dielectric constant of confined water should be different from that in bulk. Recent experiments have shown that it is vanishingly small, however the origin of the phenomenon remains unclear. Here we used ab initio molecular dynamics simulations (AIMD) and AIMD-trained machine-learning potentials to understand water's structure and electronic properties underpinning this effect. For the graphene and hexagonal boron-nitride substrates considered, we find that it originates in the spontaneous anti-parallel alignment of the water dipoles in the first two water layers near the solid interface. The interfacial layers exhibit net ferroelectric ordering, resulting in an overall anti-ferroelectric arrangement of confined water. Together with constrained hydrogen-bonding orientations, this leads to much reduced out-of-plane polarization. Furthermore, we directly contrast AIMD and simple classical force-field simulations, revealing important differences. This work offers insight into a property of water that is critical in modulating surface forces, the electric-double-layer formation and molecular solvation, and shows a way to compute it.},
keywords = {AIMD, Confinement, Hydrogen bonding, Water},
pubstate = {published},
tppubtype = {article}
}
2023
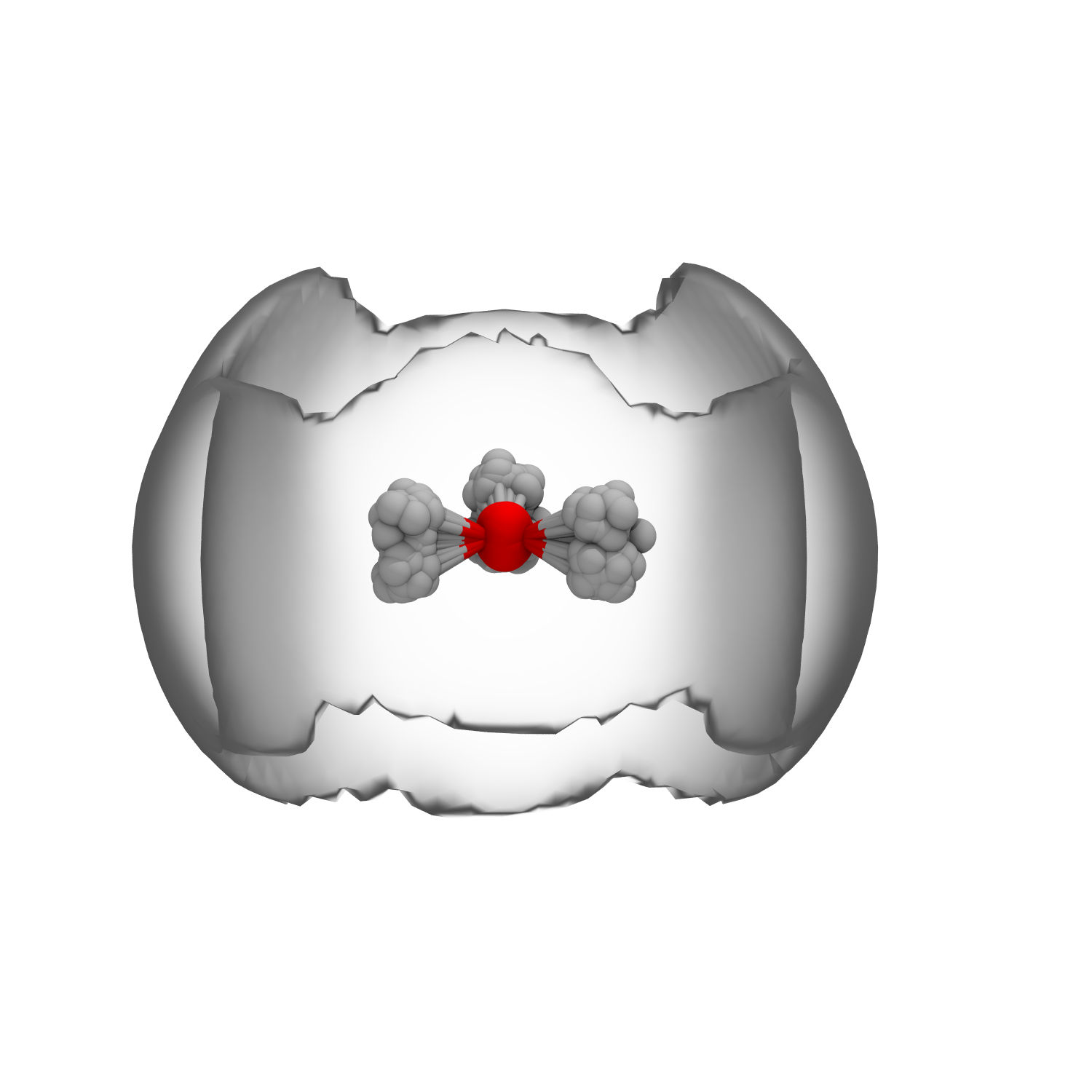
Julia A. Davies, Christoph Schran, Fabien Brieuc, Dominik Marx, Andrew M. Ellis
Onset of Rotational Decoupling for a Molecular Ion Solvated in Helium: From Tags to Rings and Shells Journal Article
In: Phys. Rev. Lett., vol. 130, iss. 8, pp. 083001, 2023.
Links | BibTeX | Tags: Nuclear quantum effects, path integral molecular dynamics (PIMD), Superfluidity, Water
@article{Schran2023/10.1103/PhysRevLett.130.083001,
title = {Onset of Rotational Decoupling for a Molecular Ion Solvated in Helium: From Tags to Rings and Shells},
author = {Julia A. Davies and Christoph Schran and Fabien Brieuc and Dominik Marx and Andrew M. Ellis},
doi = {10.1103/PhysRevLett.130.083001},
year = {2023},
date = {2023-02-01},
urldate = {2023-02-01},
journal = {Phys. Rev. Lett.},
volume = {130},
issue = {8},
pages = {083001},
publisher = {American Physical Society},
keywords = {Nuclear quantum effects, path integral molecular dynamics (PIMD), Superfluidity, Water},
pubstate = {published},
tppubtype = {article}
}
2022
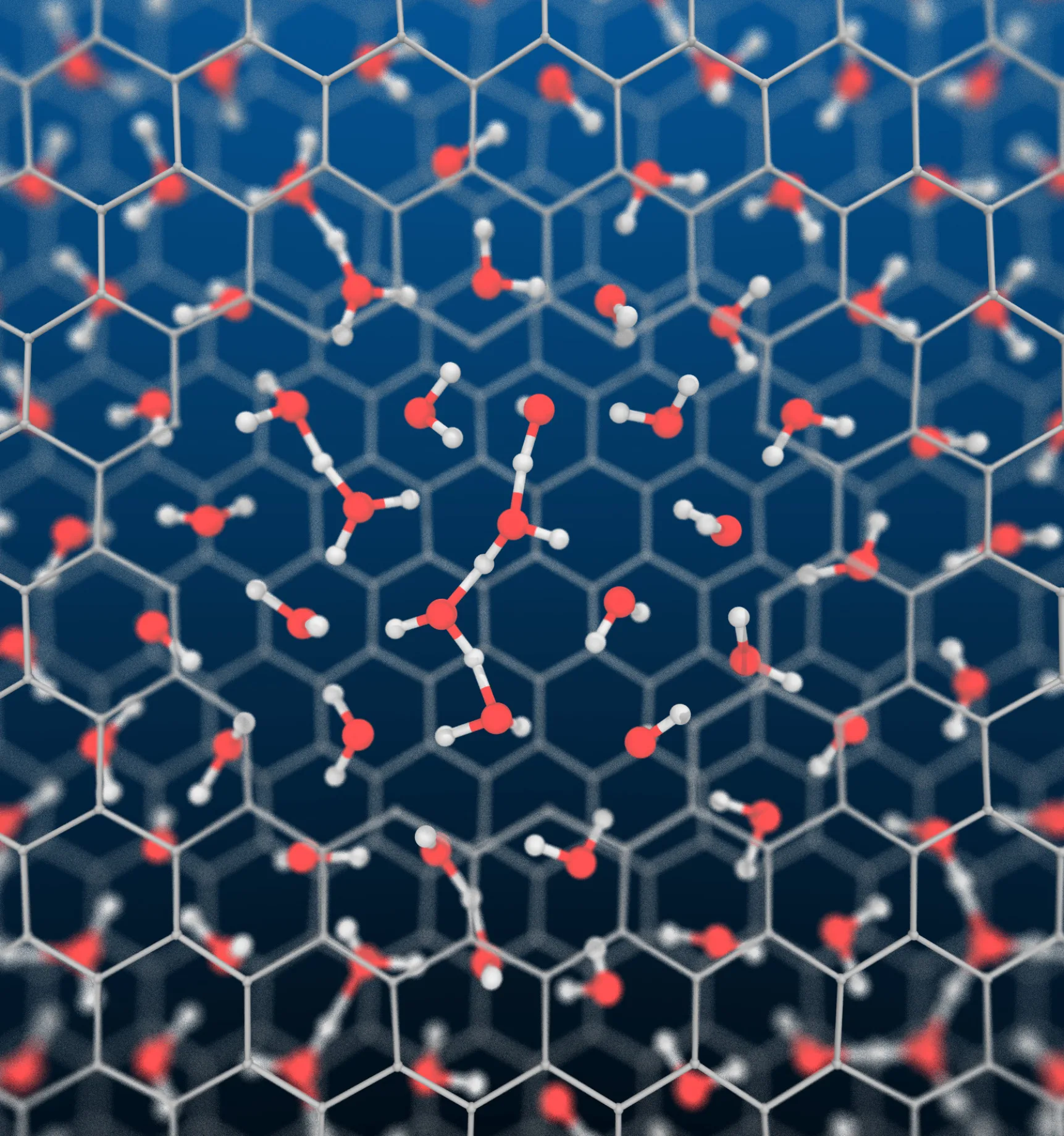
Venkat Kapil, Christoph Schran, Andrea Zen, Ji Chen, Chris J. Pickard, Angelos Michaelides
The first-principles phase diagram of monolayer nanoconfined water Journal Article
In: Nature, vol. 609, pp. 512-516, 2022, ISSN: 1476-4687.
Abstract | Links | BibTeX | Tags: Confinement, Machine Learning Potentials, Water
@article{Kapil2022/10.1038/S41586-022-05036-X,
title = {The first-principles phase diagram of monolayer nanoconfined water},
author = {Venkat Kapil and Christoph Schran and Andrea Zen and Ji Chen and Chris J. Pickard and Angelos Michaelides},
doi = {10.1038/S41586-022-05036-X},
issn = {1476-4687},
year = {2022},
date = {2022-09-01},
urldate = {2022-09-01},
journal = {Nature},
volume = {609},
pages = {512-516},
publisher = {Nature Publishing Group},
abstract = {Water in nanoscale cavities is ubiquitous and of central importance to everyday phenomena in geology and biology. However, the properties of nanoscale water can be substantially different from those of bulk water, as shown, for example, by the anomalously low dielectric constant of water in nanochannels1, near frictionless water flow2 or the possible existence of a square ice phase3. Such properties suggest that nanoconfined water could be engineered for technological applications in nanofluidics4, electrolyte materials5 and water desalination6. Unfortunately, challenges in experimentally characterizing water at the nanoscale and the high cost of first-principles simulations have prevented the molecular-level understanding required to control the behaviour of water. Here we combine a range of computational approaches to enable a first-principles-level investigation of a single layer of water within a graphene-like channel. We find that monolayer water exhibits surprisingly rich and diverse phase behaviour that is highly sensitive to temperature and the van der Waals pressure acting within the nanochannel. In addition to multiple molecular phases with melting temperatures varying non-monotonically by more than 400 kelvins with pressure, we predict a hexatic phase, which is an intermediate between a solid and a liquid, and a superionic phase with a high electrical conductivity exceeding that of battery materials. Notably, this suggests that nanoconfinement could be a promising route towards superionic behaviour under easily accessible conditions. Monolayer water exhibits rich and diverse phase behaviour that is highly sensitive to temperature and the van der Waals pressure acting within the nanochannel.},
keywords = {Confinement, Machine Learning Potentials, Water},
pubstate = {published},
tppubtype = {article}
}
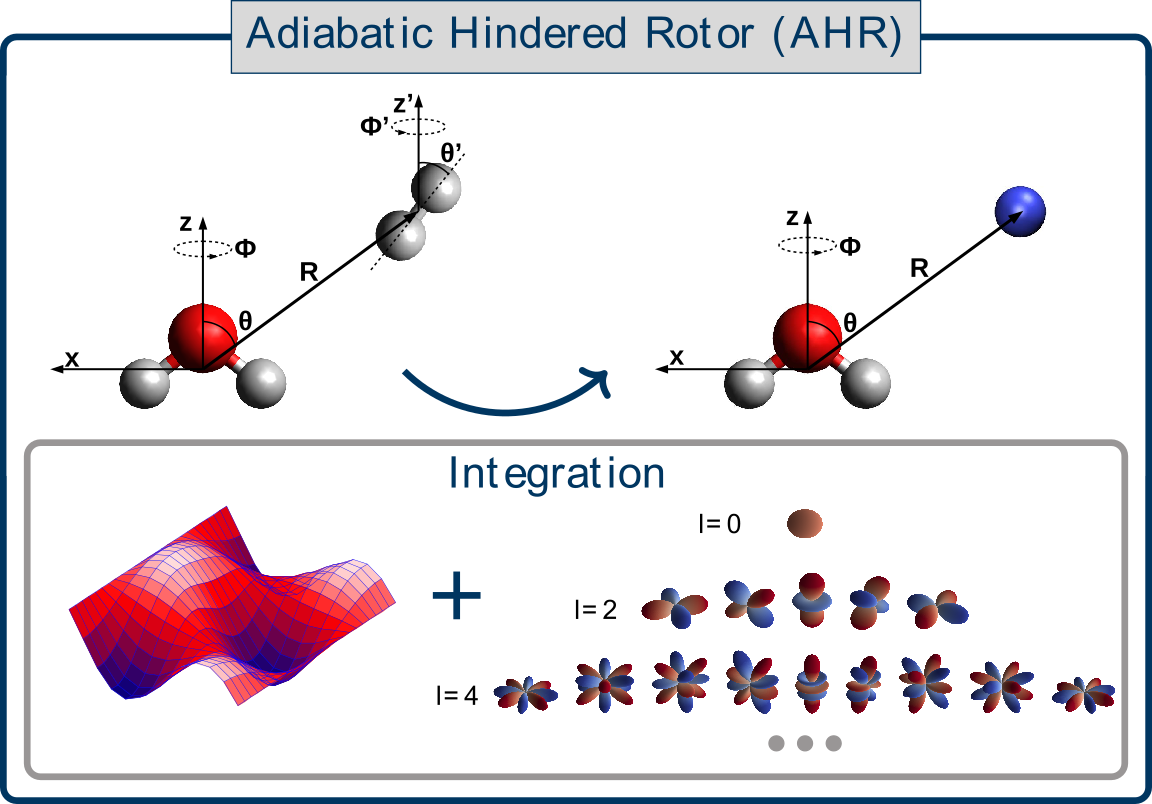
Laura Durán Caballero, Christoph Schran, Fabien Brieuc, Dominik Marx
Neural Network Interaction Potentials for para-Hydrogen with Flexible Molecules Journal Article
In: J. Chem. Phys., vol. 157, no. 7, pp. 074302, 2022, ISSN: 0021-9606.
Abstract | Links | BibTeX | Tags: Machine Learning Potentials, Nuclear quantum effects, Superfluidity, Water
@article{Duran2022/10.1063/5.0100953,
title = {Neural Network Interaction Potentials for para-Hydrogen with Flexible Molecules},
author = {Laura Durán Caballero and Christoph Schran and Fabien Brieuc and Dominik Marx},
doi = {10.1063/5.0100953},
issn = {0021-9606},
year = {2022},
date = {2022-08-01},
urldate = {2022-08-01},
journal = {J. Chem. Phys.},
volume = {157},
number = {7},
pages = {074302},
publisher = {AIP Publishing LLCAIP Publishing},
abstract = {The study of molecular impurities in para-hydrogen (pH2) clusters is key to push forward our understanding of intra- and intermolecular interactions including their impact on the superfluid response of this bosonic quantum solvent. This includes tagging with one or very few pH2, the microsolvation regime, and matrix isolation. However, the fundamental coupling between the bosonic pH2 environment and the (ro-)vibrational motion of molecular impurities remains poorly understood. Quantum simulations can in provide the necessary atomistic insight, but very accurate descriptions of the involved interactions are required. Here, we present a data-driven approach for the generation of impurity-pH2 interaction potentials based on machine learning techniques which retain the full flexibility of the impurity. We employ the well-established adiabatic hindered rotor (AHR) averaging technique to include the impact of the nuclear spin statistics on the symmetry-allowed rotational quantum numbers of pH2. Embedding this averaging procedure within the high-dimensional neural network potential (NNP) framework enables the generation of highly-accurate AHR-averaged NNPs at coupled cluster accuracy, namely CCSD(T*)-F12a/aVTZcp in an automated manner. We apply this methodology to the water and protonated water molecules, as representative cases for quasi-rigid and highly-flexible molecules respectively, and obtain AHR-averaged NNPs that reliably describe the H2O-pH2 and H3O+-pH2 interactions. Using path integral simulations we show for the hydronium cation that umbrella-like tunneling inversion has a strong impact on the first and second pH2 microsolvation shells. The data-driven nature of our protocol opens the door to the study of bosonic pH2 quantum solvation for a wide range of embedded impurities.},
keywords = {Machine Learning Potentials, Nuclear quantum effects, Superfluidity, Water},
pubstate = {published},
tppubtype = {article}
}
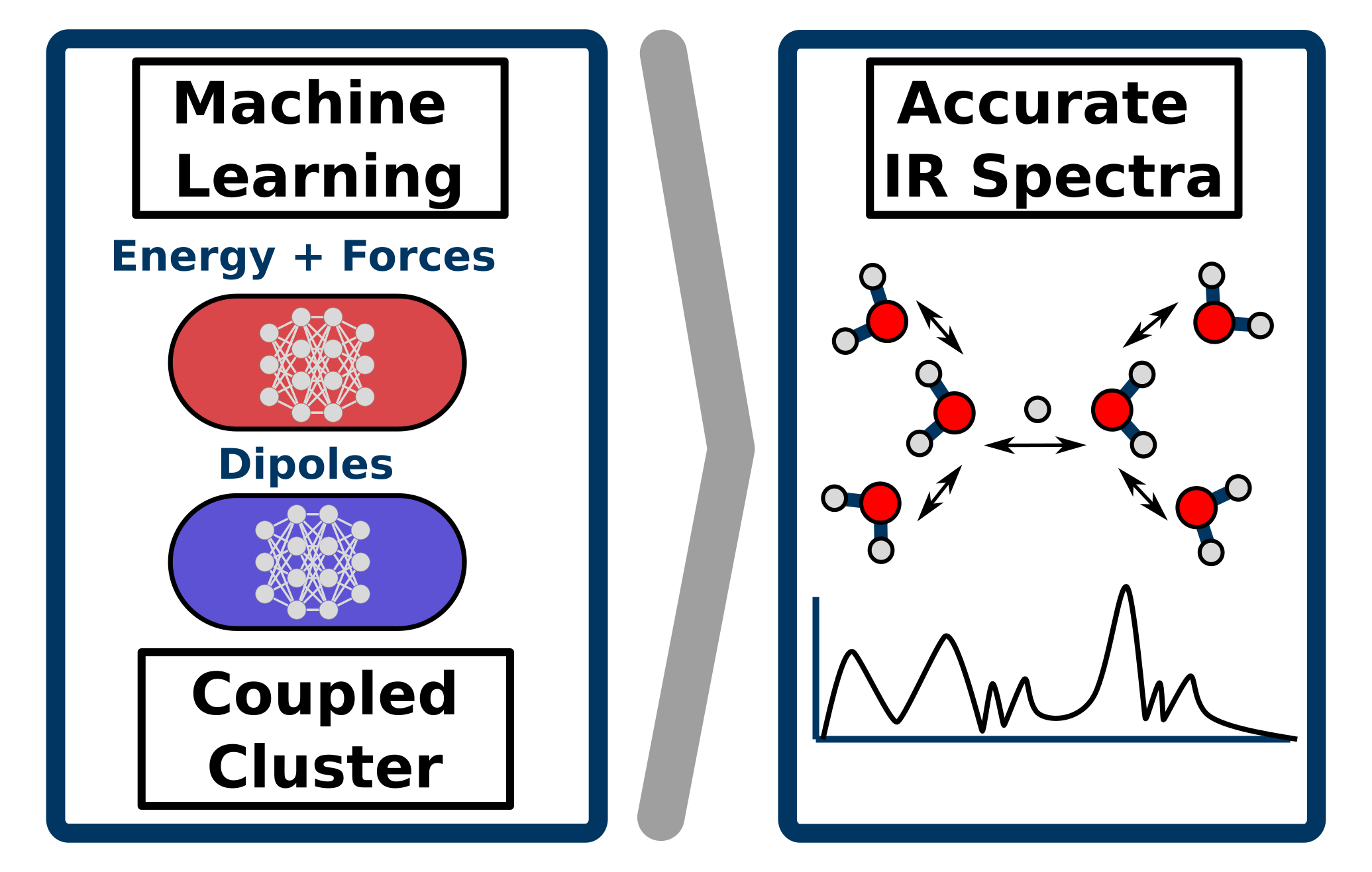
Richard Beckmann, Fabien Brieuc, Christoph Schran, Dominik Marx
Infrared spectra at coupled cluster accuracy from neural network representations Journal Article
In: J. Chem. Theory Comput., 2022, ISSN: 1549-9618.
Abstract | Links | BibTeX | Tags: Coupled Cluster, Machine Learning Potentials, Spectra, Water
@article{Beckmann2022/10.1021/ACS.JCTC.2C00511,
title = {Infrared spectra at coupled cluster accuracy from neural network representations},
author = {Richard Beckmann and Fabien Brieuc and Christoph Schran and Dominik Marx},
doi = {10.1021/ACS.JCTC.2C00511},
issn = {1549-9618},
year = {2022},
date = {2022-08-01},
urldate = {2022-08-01},
journal = {J. Chem. Theory Comput.},
publisher = {American Chemical Society},
abstract = {Infrared spectroscopy is key to elucidate molecular structures, monitor reactions and observe conformational changes, while providing information on both structural and dynamical properties. This makes the accurate prediction of infrared spectra based on first-principle theories a highly desirable pursuit. Molecular dynamics simulations have proven to be a particularly powerful approach for this task, albeit requiring the computation of energies, forces and dipole moments for a large number of molecular configurations as a function of time. This explains why highly accurate first principles methods, such as coupled cluster theory, have so far been inapplicable for the prediction of fully anharmonic vibrational spectra of large systems at finite temperatures. Here, we push cutting-edge machine learning techniques forward by using neural network representations of energies, forces and in particular dipoles to predict such infrared spectra fully at "gold standard" coupled cluster accuracy as demonstrated for protonated water clusters as large as the protonated water hexamer, in its extended Zundel configuration. Furthermore, we show that this methodology can be used beyond the scope of the data considered during the development of the neural network models, allowing for the computation of finite-temperature infrared spectra of large systems inaccessible to explicit coupled cluster calculations. This substantially expands the hitherto existing limits of accuracy, speed and system size for theoretical spectroscopy and opens up a multitude of avenues for the prediction of vibrational spectra and the understanding of complex intra- and intermolecular couplings.},
keywords = {Coupled Cluster, Machine Learning Potentials, Spectra, Water},
pubstate = {published},
tppubtype = {article}
}
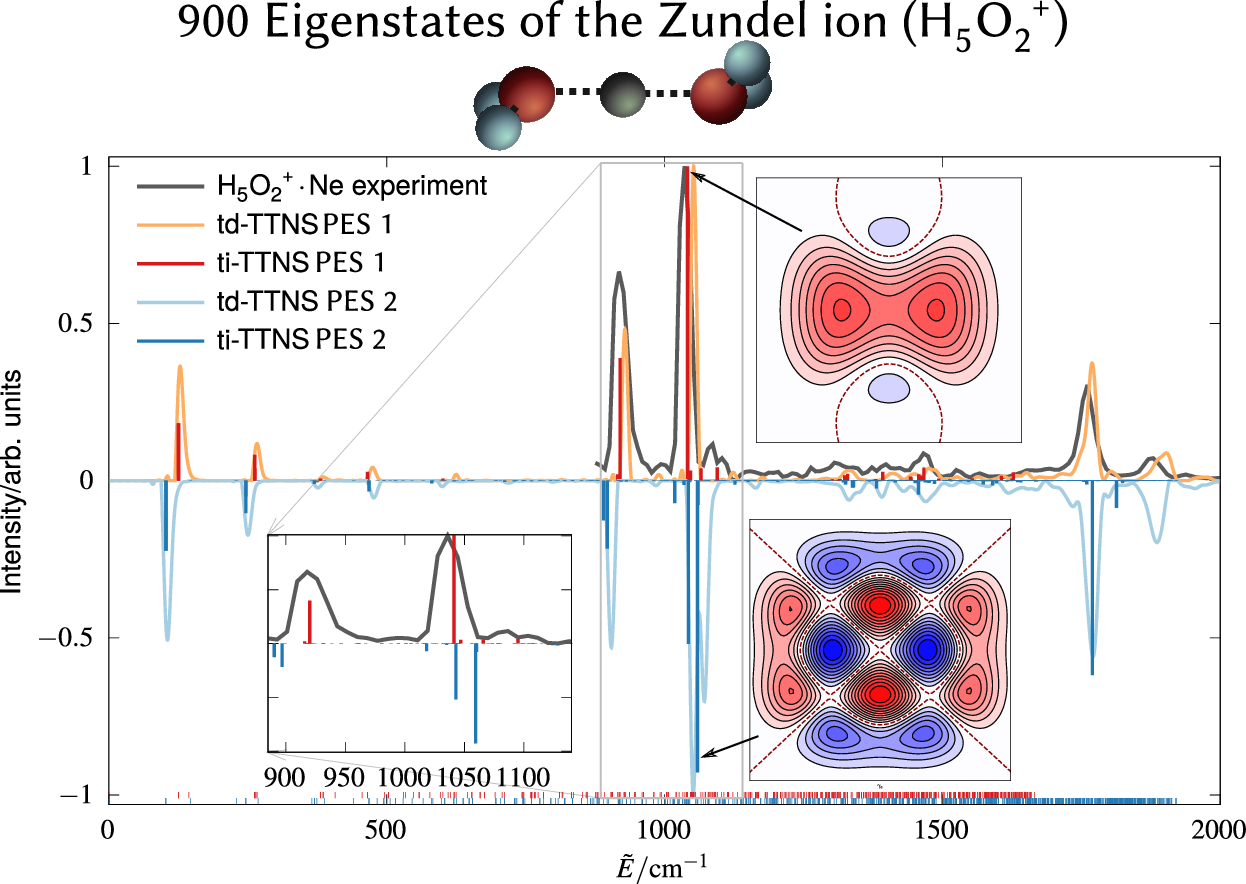
Henrik R Larsson, Markus Schröder, Richard Beckmann, Fabien Brieuc, Christoph Schran, Dominik Marx, Oriol Vendrell
State-resolved infrared spectrum of the protonated water dimer: Revisiting the characteristic proton transfer doublet peak Journal Article
In: Chem. Sci., 2022, ISSN: 2041-6539.
Abstract | Links | BibTeX | Tags: Quantum Dynamics, Spectra, Water
@article{Larsson2022/10.1039/D2SC03189B,
title = {State-resolved infrared spectrum of the protonated water dimer: Revisiting the characteristic proton transfer doublet peak},
author = {Henrik R Larsson and Markus Schröder and Richard Beckmann and Fabien Brieuc and Christoph Schran and Dominik Marx and Oriol Vendrell},
doi = {10.1039/D2SC03189B},
issn = {2041-6539},
year = {2022},
date = {2022-08-01},
urldate = {2022-08-01},
journal = {Chem. Sci.},
publisher = {The Royal Society of Chemistry},
abstract = {The infrared (IR) spectra of protonated water clusters encode precise information on the dynamics and structure of the hydrated proton. However, the strong anharmonic coupling and quantum effects of these elusive species remain puzzling up to the present day. Here, we report unequivocal evidence that the interplay between the proton transfer and the water wagging motions in the protonated water dimer (Zundel ion) giving rise to the characteristic doublet peak is both more complex and more sensitive to subtle energetic changes than previously thought. In particular, hitherto overlooked low-intensity satellite peaks in the experimental spectrum are now unveiled and mechanistically assigned. Our findings rely on the comparison of IR spectra obtained using two highly accurate potential energy surfaces in conjunction with highly accurate state-resolved quantum simulations. We demonstrate that these high-accuracy simulations are important for providing definite assignments of the complex IR signals of fluxional molecules.},
keywords = {Quantum Dynamics, Spectra, Water},
pubstate = {published},
tppubtype = {article}
}
2021
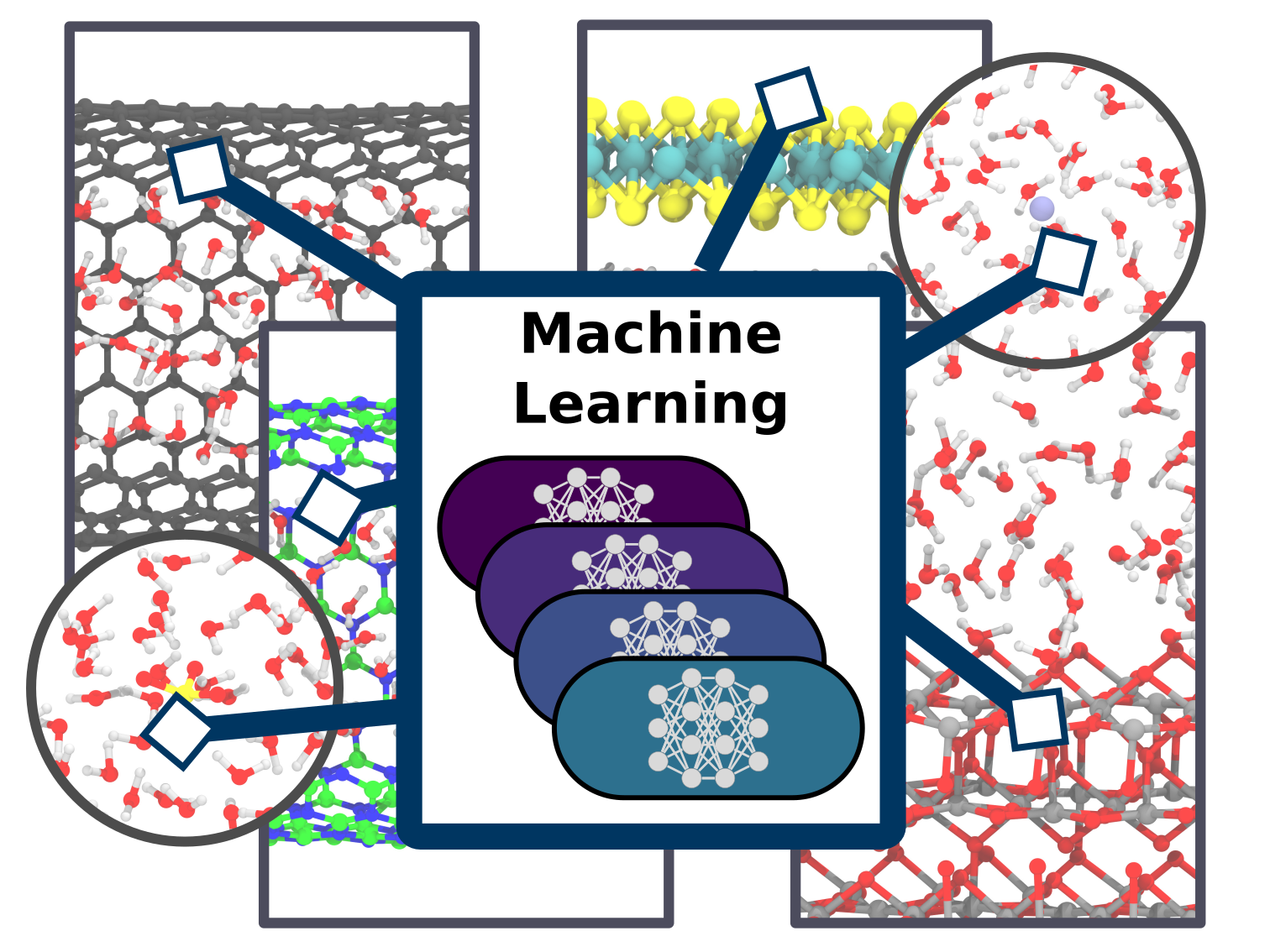
Christoph Schran, Fabian L. Thiemann, Patrick Rowe, Erich A. Müller, Ondrej Marsalek, Angelos Michaelides
Machine learning potentials for complex aqueous systems made simple Journal Article
In: Proc. Natl. Acad. Sci., vol. 118, no. 38, pp. e2110077118, 2021, ISSN: 0027-8424.
Abstract | Links | BibTeX | Tags: Confinement, Ions in Water, Machine Learning Potentials, Water, Water at Interfaces
@article{Schran2021/10.1073/PNAS.2110077118,
title = {Machine learning potentials for complex aqueous systems made simple},
author = {Christoph Schran and Fabian L. Thiemann and Patrick Rowe and Erich A. Müller and Ondrej Marsalek and Angelos Michaelides},
doi = {10.1073/PNAS.2110077118},
issn = {0027-8424},
year = {2021},
date = {2021-09-01},
urldate = {2021-09-01},
journal = {Proc. Natl. Acad. Sci.},
volume = {118},
number = {38},
pages = {e2110077118},
publisher = {National Academy of Sciences},
abstract = {Simulation techniques based on accurate and efficient representations of potential energy surfaces are urgently needed for the understanding of complex aqueous systems such as solid-liquid interfaces. Here, we present a machine learning framework that enables the efficient development and validation of models for complex aqueous systems. Instead of trying to deliver a globally-optimal machine learning potential, we propose to develop models applicable to specific thermodynamic state points in a simple and user-friendly process. After an initial ab initio simulation, a machine learning potential is constructed with minimum human effort through a data-driven active learning protocol. Such models can afterwards be applied in exhaustive simulations to provide reliable answers for the scientific question at hand. We showcase this methodology on a diverse set of aqueous systems with increasing degrees of complexity. The systems chosen here comprise water with different ions in solution, water on a titanium dioxide surface, as well as water confined in nanotubes and between molybdenum disulfide sheets. Highlighting the accuracy of our approach compared to the ab initio reference, the resulting models are evaluated in detail with an automated validation protocol that includes structural and dynamical properties and the precision of the force prediction of the models. Finally, we demonstrate the capabilities of our approach for the description of water on the rutile titanium dioxide (110) surface to analyze structure and mobility of water on this surface. Such machine learning models provide a straightforward and uncomplicated but accurate extension of simulation time and length scales for complex systems.},
keywords = {Confinement, Ions in Water, Machine Learning Potentials, Water, Water at Interfaces},
pubstate = {published},
tppubtype = {article}
}
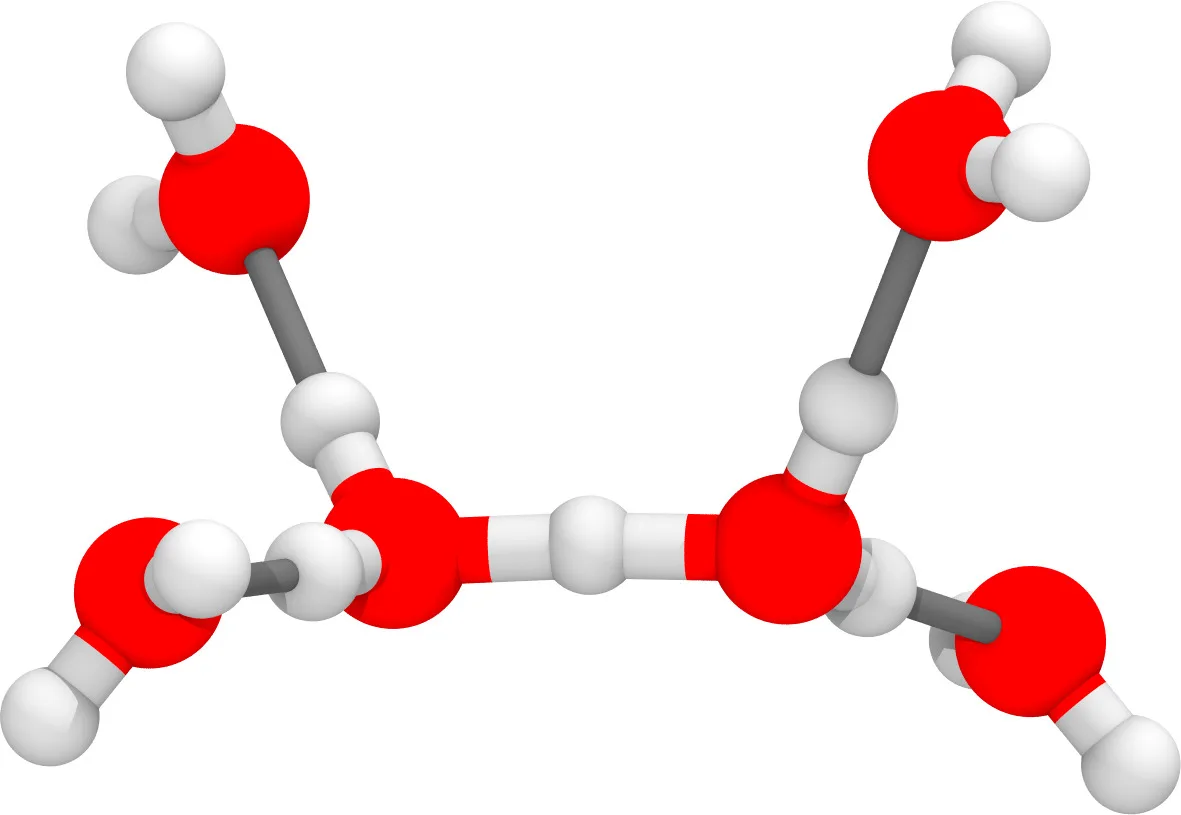
Christoph Schran, Fabien Brieuc, Dominik Marx
Transferability of machine learning potentials: Protonated water neural network potential applied to the protonated water hexamer Journal Article
In: J. Chem. Phys., vol. 154, no. 5, pp. 051101, 2021, ISSN: 10897690.
Abstract | Links | BibTeX | Tags: Coupled Cluster, Hydrogen bonding, Machine Learning Potentials, Water
@article{Schran2021/10.1063/5.0035438,
title = {Transferability of machine learning potentials: Protonated water neural network potential applied to the protonated water hexamer},
author = {Christoph Schran and Fabien Brieuc and Dominik Marx},
doi = {10.1063/5.0035438},
issn = {10897690},
year = {2021},
date = {2021-02-01},
urldate = {2021-02-01},
journal = {J. Chem. Phys.},
volume = {154},
number = {5},
pages = {051101},
abstract = {A previously published neural network potential for the description of protonated water clusters up to the protonated water tetramer, H+(H2O)4, at an essentially converged coupled cluster accuracy [C. Schran, J. Behler, and D. Marx, J. Chem. Theory Comput. 16, 88 (2020)] is applied to the protonated water hexamer, H+(H2O)6 - a system that the neural network has never seen before. Although being in the extrapolation regime, it is shown that the potential not only allows for quantum simulations from ultra-low temperatures ∼1 K up to 300 K but is also able to describe the new system very accurately compared to explicit coupled cluster calculations. This transferability of the model is rationalized by the similarity of the atomic environments encountered for the larger cluster compared to the environments in the training set of the model. Compared to the interpolation regime, the quality of the model is reduced by roughly one order of magnitude, but most of the difference to the coupled cluster reference comes from global shifts of the potential energy surface, while local energy fluctuations are well recovered. These results suggest that the application of neural network potentials in extrapolation regimes can provide useful results and might be more general than usually thought.},
keywords = {Coupled Cluster, Hydrogen bonding, Machine Learning Potentials, Water},
pubstate = {published},
tppubtype = {article}
}
2020
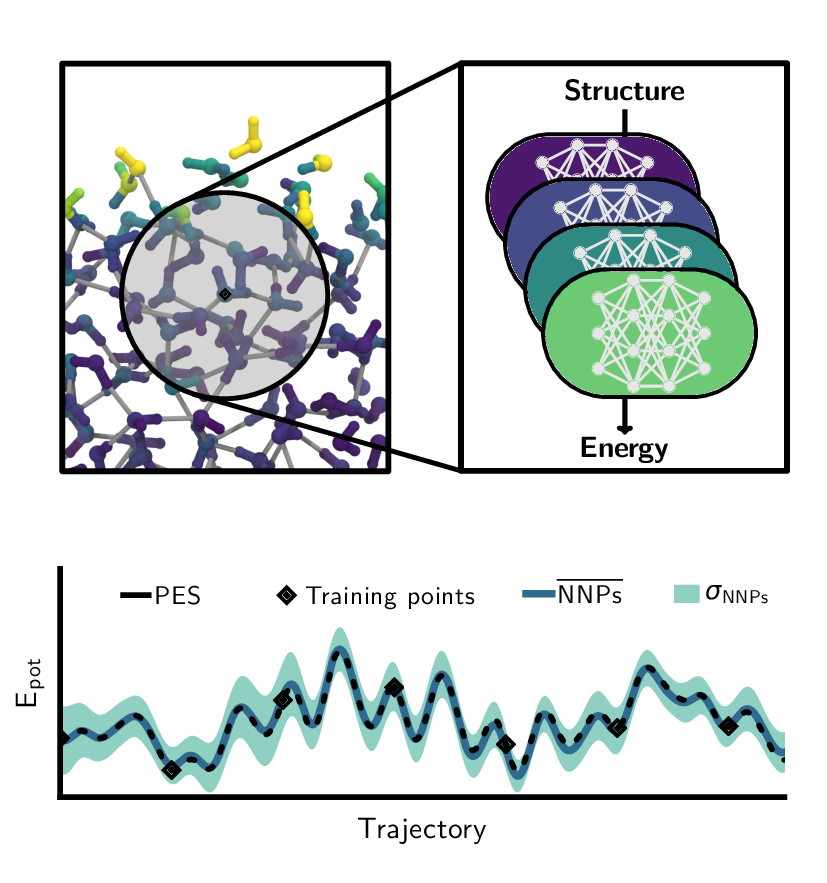
Christoph Schran, Krystof Brezina, Ondrej Marsalek
Committee neural network potentials control generalization errors and enable active learning Journal Article
In: J. Chem. Phys., vol. 153, no. 10, pp. 104105, 2020, ISSN: 10897690.
Abstract | Links | BibTeX | Tags: Machine Learning Potentials, path integral molecular dynamics (PIMD), Water
@article{Schran2020/10.1063/5.0016004,
title = {Committee neural network potentials control generalization errors and enable active learning},
author = {Christoph Schran and Krystof Brezina and Ondrej Marsalek},
doi = {10.1063/5.0016004},
issn = {10897690},
year = {2020},
date = {2020-09-01},
urldate = {2020-09-01},
journal = {J. Chem. Phys.},
volume = {153},
number = {10},
pages = {104105},
abstract = {It is well known in the field of machine learning that committee models improve accuracy, provide generalization error estimates, and enable active learning strategies. In this work, we adapt these concepts to interatomic potentials based on artificial neural networks. Instead of a single model, multiple models that share the same atomic environment descriptors yield an average that outperforms its individual members as well as a measure of the generalization error in the form of the committee disagreement. We not only use this disagreement to identify the most relevant configurations to build up the model's training set in an active learning procedure but also monitor and bias it during simulations to control the generalization error. This facilitates the adaptive development of committee neural network potentials and their training sets while keeping the number of ab initio calculations to a minimum. To illustrate the benefits of this methodology, we apply it to the development of a committee model for water in the condensed phase. Starting from a single reference ab initio simulation, we use active learning to expand into new state points and to describe the quantum nature of the nuclei. The final model, trained on 814 reference calculations, yields excellent results under a range of conditions, from liquid water at ambient and elevated temperatures and pressures to different phases of ice, and the air-water interface - all including nuclear quantum effects. This approach to committee models will enable the systematic development of robust machine learning models for a broad range of systems.},
keywords = {Machine Learning Potentials, path integral molecular dynamics (PIMD), Water},
pubstate = {published},
tppubtype = {article}
}
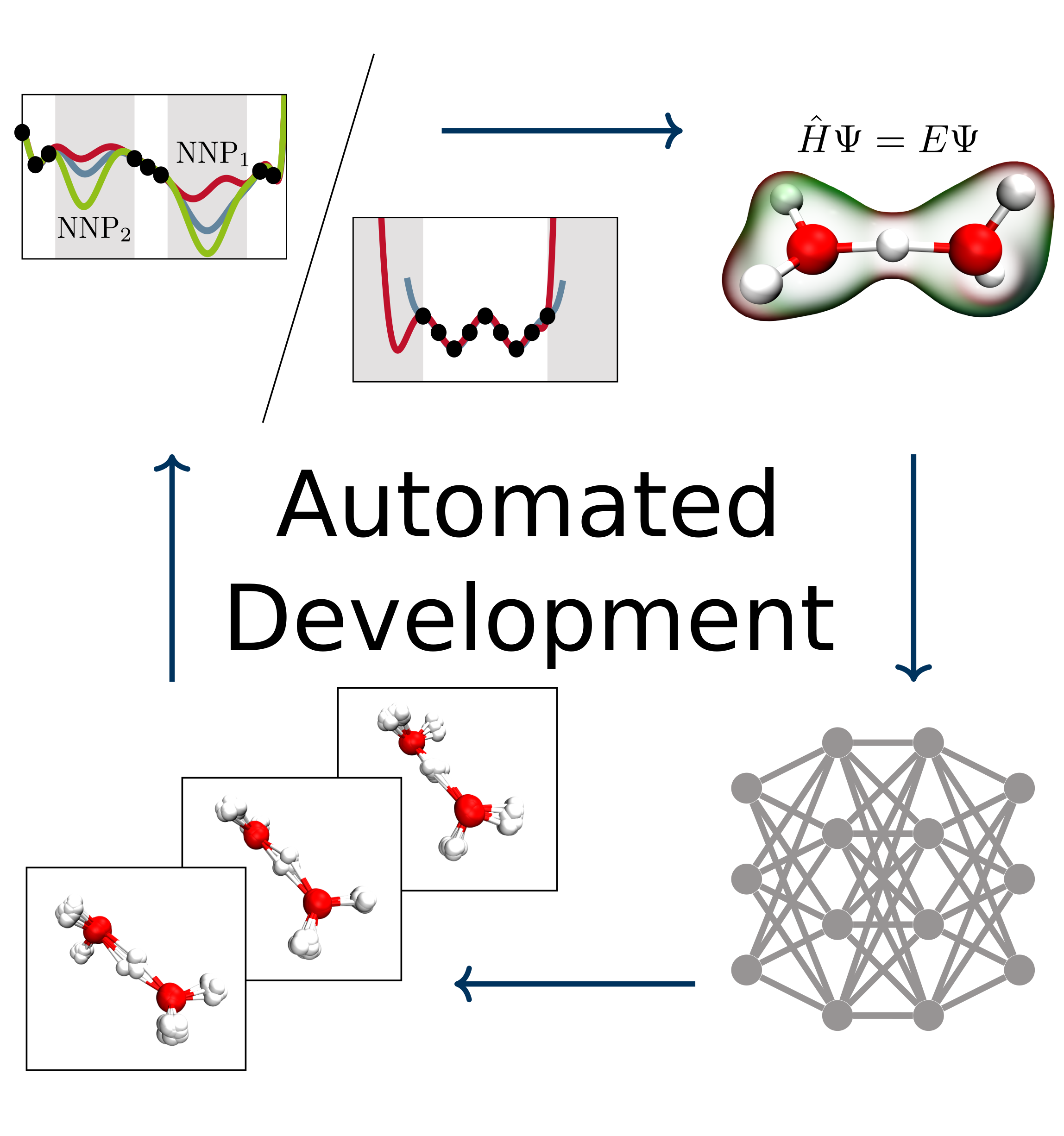
Christoph Schran, Jörg Behler, Dominik Marx
Automated Fitting of Neural Network Potentials at Coupled Cluster Accuracy: Protonated Water Clusters as Testing Ground Journal Article
In: J. Chem. Theory Comput., vol. 16, no. 1, pp. 88–99, 2020, ISSN: 15499626.
Abstract | Links | BibTeX | Tags: Coupled Cluster, Hydrogen bonding, Machine Learning Potentials, Water
@article{Schran2020/10.1021/acs.jctc.9b00805,
title = {Automated Fitting of Neural Network Potentials at Coupled Cluster Accuracy: Protonated Water Clusters as Testing Ground},
author = {Christoph Schran and Jörg Behler and Dominik Marx},
doi = {10.1021/acs.jctc.9b00805},
issn = {15499626},
year = {2020},
date = {2020-01-01},
urldate = {2020-01-01},
journal = {J. Chem. Theory Comput.},
volume = {16},
number = {1},
pages = {88–99},
abstract = {Highly accurate potential energy surfaces are of key interest for the detailed understanding and predictive modeling of chemical systems. In recent years, several new types of force fields, which are based on machine learning algorithms and fitted to ab initio reference calculations, have been introduced to meet this requirement. Here, we show how high-dimensional neural network potentials can be employed to automatically generate the potential energy surface of finite sized clusters at coupled cluster accuracy, namely CCSD(T*)-F12a/aug-cc-pVTZ. The developed automated procedure utilizes the established intrinsic properties of the model such that the configurations for the training set are selected in an unbiased and efficient way to minimize the computational effort of expensive reference calculations. These ideas are applied to protonated water clusters from the hydronium cation, H3O+, up to the tetramer, H9O4+, and lead to a single potential energy surface that describes all these systems at essentially converged coupled cluster accuracy with a fitting error of 0.06 kJ/mol per atom. The fit is validated in detail for all clusters up to the tetramer and yields reliable results not only for stationary points but also for reaction pathways and intermediate configurations as well as different sampling techniques. Per design, the neural network potentials (NNPs) constructed in this fashion can handle very different conditions including the quantum nature of the nuclei and enhanced sampling techniques covering very low as well as high temperatures. This enables fast and exhaustive exploration of the targeted protonated water clusters with essentially converged interactions. In addition, the automated process will allow one to tackle finite systems much beyond the present case.},
keywords = {Coupled Cluster, Hydrogen bonding, Machine Learning Potentials, Water},
pubstate = {published},
tppubtype = {article}
}
2019
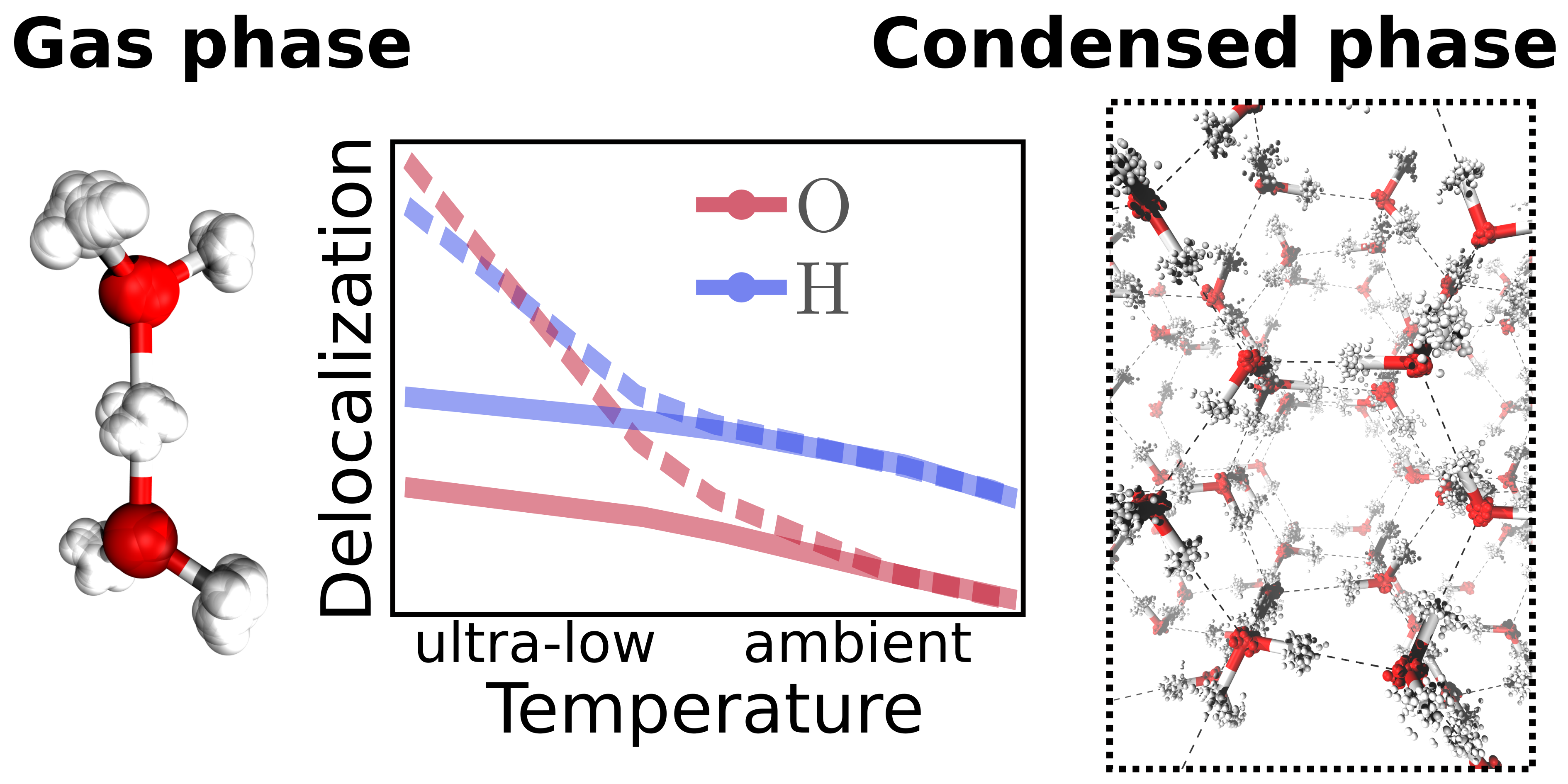
Christoph Schran, Dominik Marx
Quantum nature of the hydrogen bond from ambient conditions down to ultra-low temperatures Journal Article
In: Phys. Chem. Chem. Phys., vol. 21, no. 45, pp. 24967–24975, 2019, ISSN: 14639076.
Abstract | Links | BibTeX | Tags: Hydrogen bonding, Nuclear quantum effects, path integral molecular dynamics (PIMD), Water
@article{Schran2019/10.1039/C9CP04795F,
title = {Quantum nature of the hydrogen bond from ambient conditions down to ultra-low temperatures},
author = {Christoph Schran and Dominik Marx},
doi = {10.1039/c9cp04795f},
issn = {14639076},
year = {2019},
date = {2019-10-01},
urldate = {2019-10-01},
journal = {Phys. Chem. Chem. Phys.},
volume = {21},
number = {45},
pages = {24967–24975},
abstract = {Many experimental techniques such as tagging photodissociation and helium nanodroplet isolation spectroscopy operate at very low temperatures in order to investigate hydrogen bonding. To elucidate the differences between such ultra-cold and usual ambient conditions, different hydrogen bonded systems are studied systematically from 300 K down to about 1 K using path integral simulations that explicitly consider both the quantum nature of the nuclei and thermal fluctuations. For this purpose, finite sized water clusters, specifically the water dimer and hexamer, protonated water clusters including the Zundel and Eigen complexes, as well as hexagonal ice as a condensed phase representative are compared directly as a function of temperature. While weaker hydrogen bonds, as present in the neutral systems, show distinct structural differences between ambient conditions and the ultra-cold regime, the stronger hydrogen bonds of the protonated water clusters are less perturbed by temperature compared to their quantum ground state. In all the studied systems, the quantum delocalization of the nuclei is found to vary drastically with temperature. Interestingly, upon reaching temperatures of about 1 K, the spatial quantum delocalization of the heavy oxygens approaches that of the protons for relatively weak spatial constraints, and even significantly exceeds the latter in the case of the centered hydrogen bond in the Zundel complex. These findings are relevant for comparisons between experiments on hydrogen bonding carried out under ultra-cold versus ambient conditions as well as to understand quantum delocalization phenomena of nuclei by seamlessly extending our insights into noncovalent interactions down to ultra-low temperatures.},
keywords = {Hydrogen bonding, Nuclear quantum effects, path integral molecular dynamics (PIMD), Water},
pubstate = {published},
tppubtype = {article}
}
2018
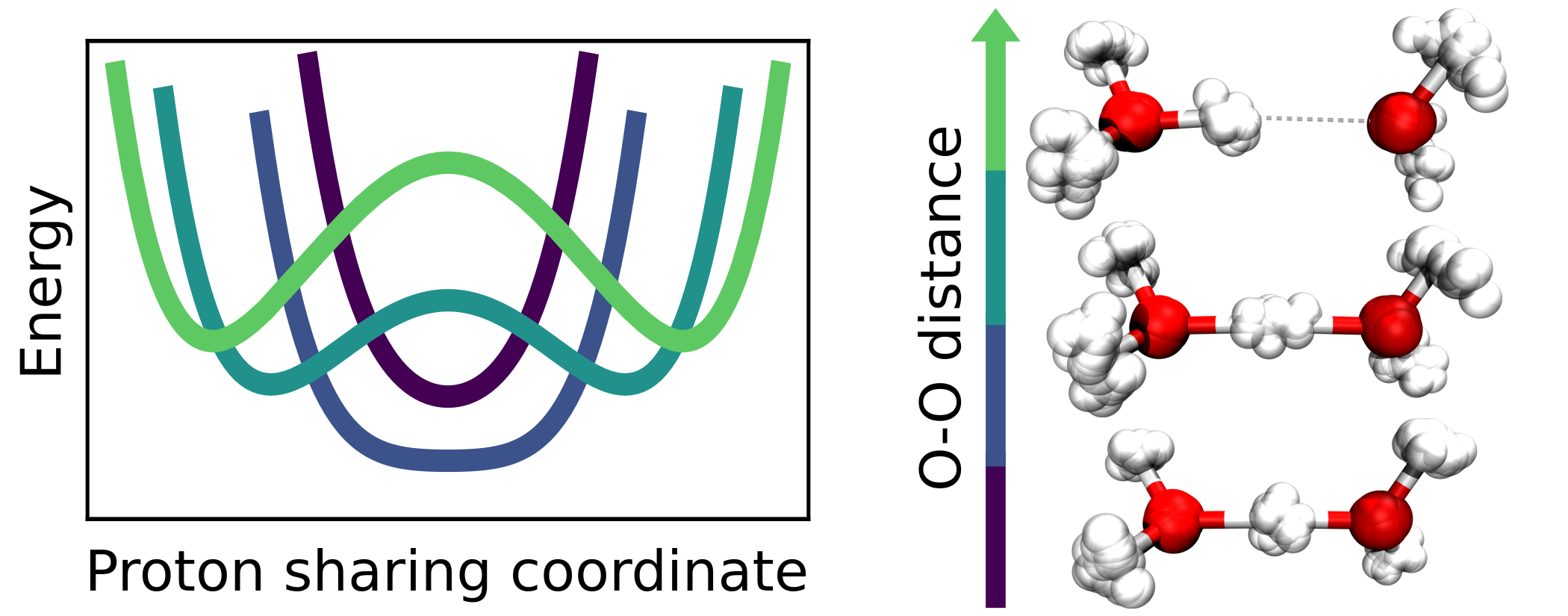
Christoph Schran, Fabien Brieuc, Dominik Marx
Converged Colored Noise Path Integral Molecular Dynamics Study of the Zundel Cation Down to Ultralow Temperatures at Coupled Cluster Accuracy Journal Article
In: J. Chem. Theory Comput., vol. 14, no. 10, pp. 5068–5078, 2018, ISSN: 15499626.
Abstract | Links | BibTeX | Tags: Nuclear quantum effects, path integral molecular dynamics (PIMD), Water
@article{Schran2018/10.1021/acs.jctc.8b00705,
title = {Converged Colored Noise Path Integral Molecular Dynamics Study of the Zundel Cation Down to Ultralow Temperatures at Coupled Cluster Accuracy},
author = {Christoph Schran and Fabien Brieuc and Dominik Marx},
doi = {10.1021/acs.jctc.8b00705},
issn = {15499626},
year = {2018},
date = {2018-09-01},
urldate = {2018-09-01},
journal = {J. Chem. Theory Comput.},
volume = {14},
number = {10},
pages = {5068–5078},
abstract = {For a long time, performing converged path integral simulations at ultra-low, but finite temperatures of a few Kelvin has been a nearly impossible task. However, recent developments in advanced colored noise thermostatting schemes for path integral simulations, namely the Path Integral Generalized Langevin Equation Thermostat (PIGLET) and the Path Integral Quantum Thermal Bath (PIQTB), have been able to greatly reduce the computational cost of these simulations, thus making the ultra-low temperature regime accessible in practice. In this work, we investigate the influence of these two thermostatting schemes on the description of hydrogen-bonded systems at temperatures down to a few Kelvin as encountered, for example, in helium nanodroplet isolation or tagging photodissociation spectroscopy experiments. For this purpose, we analyze the prototypical hydrogen bond in the Zundel cation (H5O2+) as a function of both, oxygen-oxygen distance and temperature in order to elucidate how the anisotropic quantum deloc...},
keywords = {Nuclear quantum effects, path integral molecular dynamics (PIMD), Water},
pubstate = {published},
tppubtype = {article}
}
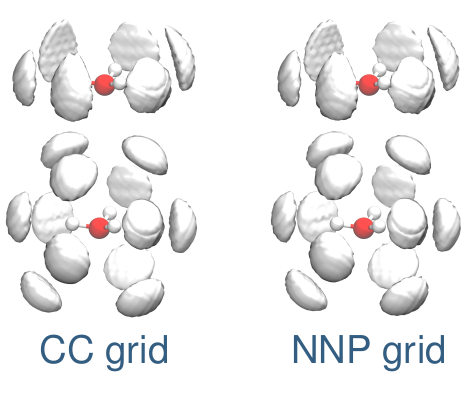
Christoph Schran, Felix Uhl, Jörg Behler, Dominik Marx
High-dimensional neural network potentials for solvation: The case of protonated water clusters in helium Journal Article
In: J. Chem. Phys., vol. 148, no. 10, pp. 102310, 2018, ISSN: 00219606.
Abstract | Links | BibTeX | Tags: Machine Learning Potentials, Nuclear quantum effects, Superfluidity, Water
@article{Schran2018/10.1063/1.4996819,
title = {High-dimensional neural network potentials for solvation: The case of protonated water clusters in helium},
author = {Christoph Schran and Felix Uhl and Jörg Behler and Dominik Marx},
doi = {10.1063/1.4996819},
issn = {00219606},
year = {2018},
date = {2018-03-01},
urldate = {2018-03-01},
journal = {J. Chem. Phys.},
volume = {148},
number = {10},
pages = {102310},
abstract = {The design of accurate helium-solute interaction potentials for the simulation of chemically complex molecules solvated in superfluid helium has long been a cumbersome task due to the rather weak but strongly anisotropic nature of the interactions. We show that this challenge can be met by using a combination of an effective pair potential for the He–He interactions and a flexible high-dimensional neural network potential (NNP) for describing the complex interaction between helium and the solute in a pairwise additive manner. This approach yields an excellent agreement with a mean absolute deviation as small as 0.04 kJ mol−1 for the interaction energy between helium and both hydronium and Zundel cations compared with coupled cluster reference calculations with an energetically converged basis set. The construction and improvement of the potential can be performed in a highly automated way, which opens the door for applications to a variety of reactive molecules to study the effect of solvation on the solu...},
keywords = {Machine Learning Potentials, Nuclear quantum effects, Superfluidity, Water},
pubstate = {published},
tppubtype = {article}
}
2017
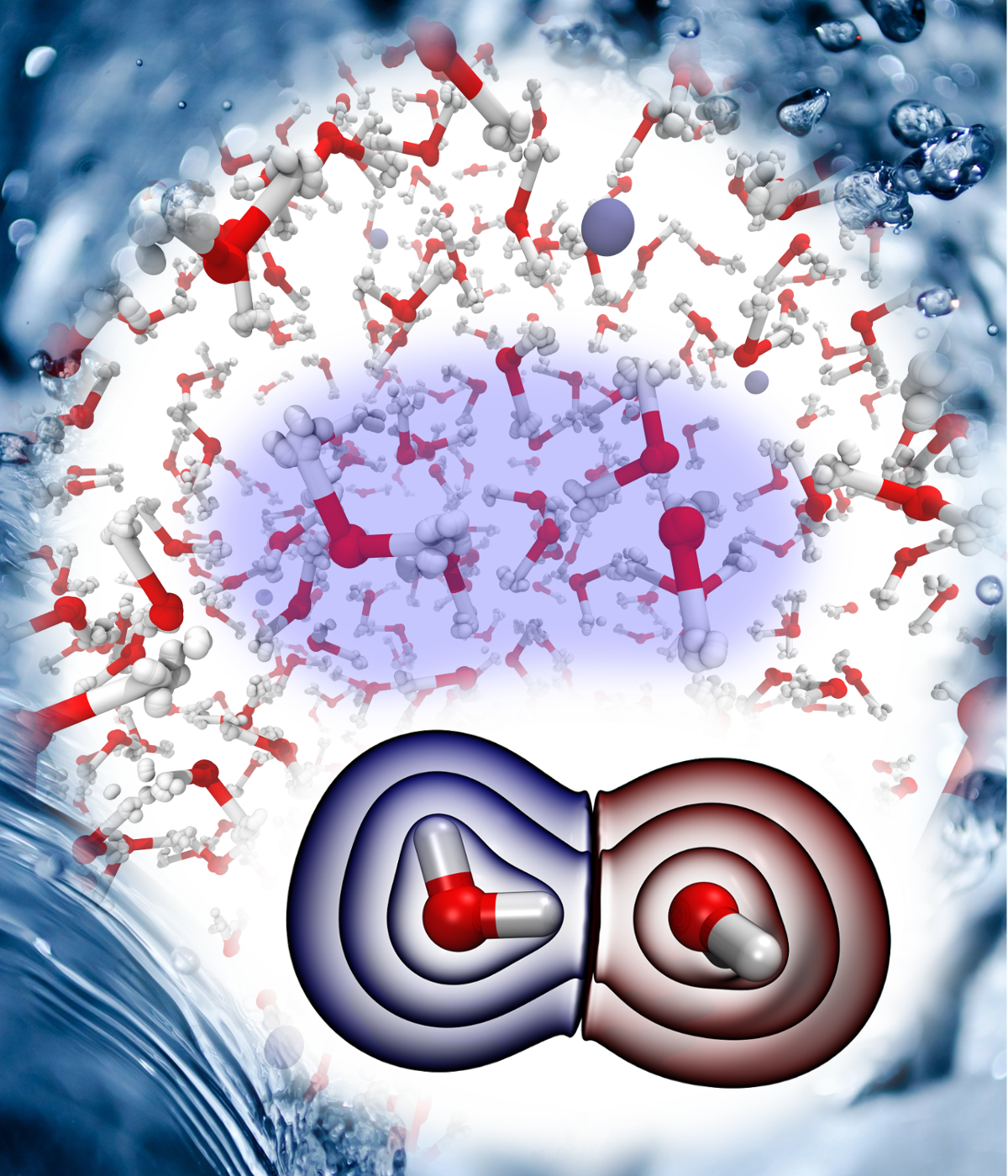
Christoph Schran, Ondrej Marsalek, Thomas E. Markland
Unravelling the influence of quantum proton delocalization on electronic charge transfer through the hydrogen bond Journal Article
In: Chem. Phys. Lett., vol. 678, pp. 289–295, 2017, ISSN: 00092614.
Abstract | Links | BibTeX | Tags: Charge transfer, Hydrogen bonding, Ions in Water, Nuclear quantum effects, Water
@article{Schran2017/10.1016/j.cplett.2017.04.034,
title = {Unravelling the influence of quantum proton delocalization on electronic charge transfer through the hydrogen bond},
author = {Christoph Schran and Ondrej Marsalek and Thomas E. Markland},
doi = {10.1016/j.cplett.2017.04.034},
issn = {00092614},
year = {2017},
date = {2017-06-01},
urldate = {2017-06-01},
journal = {Chem. Phys. Lett.},
volume = {678},
pages = {289–295},
abstract = {Upon hydrogen bond formation, electronic charge density is transferred between the donor and acceptor, impacting processes ranging from hydration to spectroscopy. Here we use ab initio path integral simulations to elucidate the role of nuclear quantum effects in determining the charge transfer in a range of hydrogen bonded species in the gas and liquid phase. We show that the quantization of the nuclei gives rise to large changes in the magnitude of the charge transfer as well as its temperature dependence. We then explain how a single geometric parameter determines the charge transfer through the hydrogen bond. These results thus demonstrate that nuclear quantum effects are vital for the accurate description of charge transfer and offer a physically transparent way to understand how hydrogen bonding gives rise to it.},
keywords = {Charge transfer, Hydrogen bonding, Ions in Water, Nuclear quantum effects, Water},
pubstate = {published},
tppubtype = {article}
}
2016
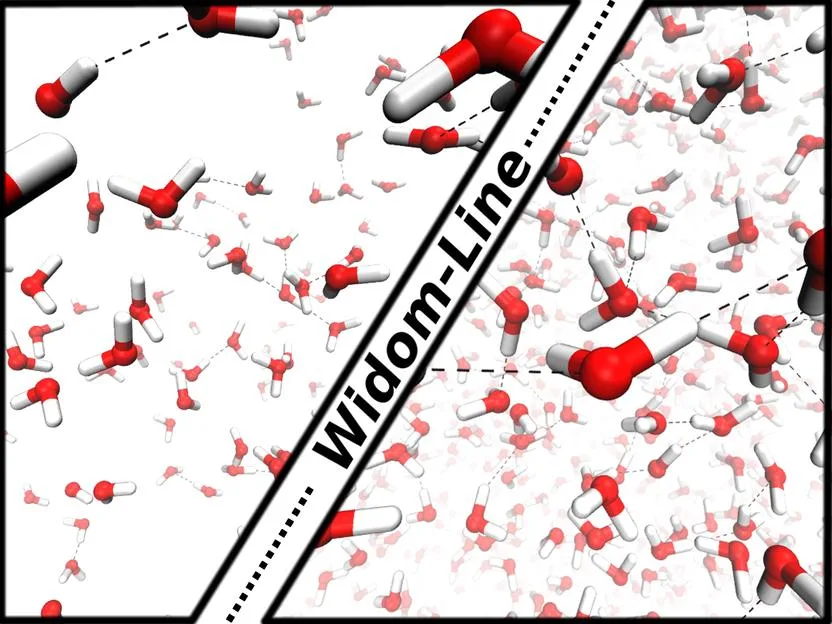
Maciej Śmiechowski, Christoph Schran, Harald Forbert, Dominik Marx
Correlated Particle Motion and THz Spectral Response of Supercritical Water Journal Article
In: Phys. Rev. Lett., vol. 116, no. 2, 2016, ISSN: 10797114.
Abstract | Links | BibTeX | Tags: Hydrogen bonding, Spectra, Water
@article{Smiechowski2016/10.1103/PhysRevLett.116.027801,
title = {Correlated Particle Motion and THz Spectral Response of Supercritical Water},
author = {Maciej Śmiechowski and Christoph Schran and Harald Forbert and Dominik Marx},
doi = {10.1103/PhysRevLett.116.027801},
issn = {10797114},
year = {2016},
date = {2016-01-01},
urldate = {2016-01-01},
journal = {Phys. Rev. Lett.},
volume = {116},
number = {2},
abstract = {Molecular dynamics simulations of supercritical water reveal distinctly different distance-dependent modulations of dipolar response and correlations in particle motion compared to ambient conditions. The strongly perturbed H-bond network of water at supercritical conditions allows for considerable translational and rotational freedom of individual molecules. These changes give rise to substantially different infrared spectra and vibrational density of states at THz frequencies for densities above and below the Widom line that separates percolating liquidlike and clustered gaslike supercritical water.},
keywords = {Hydrogen bonding, Spectra, Water},
pubstate = {published},
tppubtype = {article}
}