2024
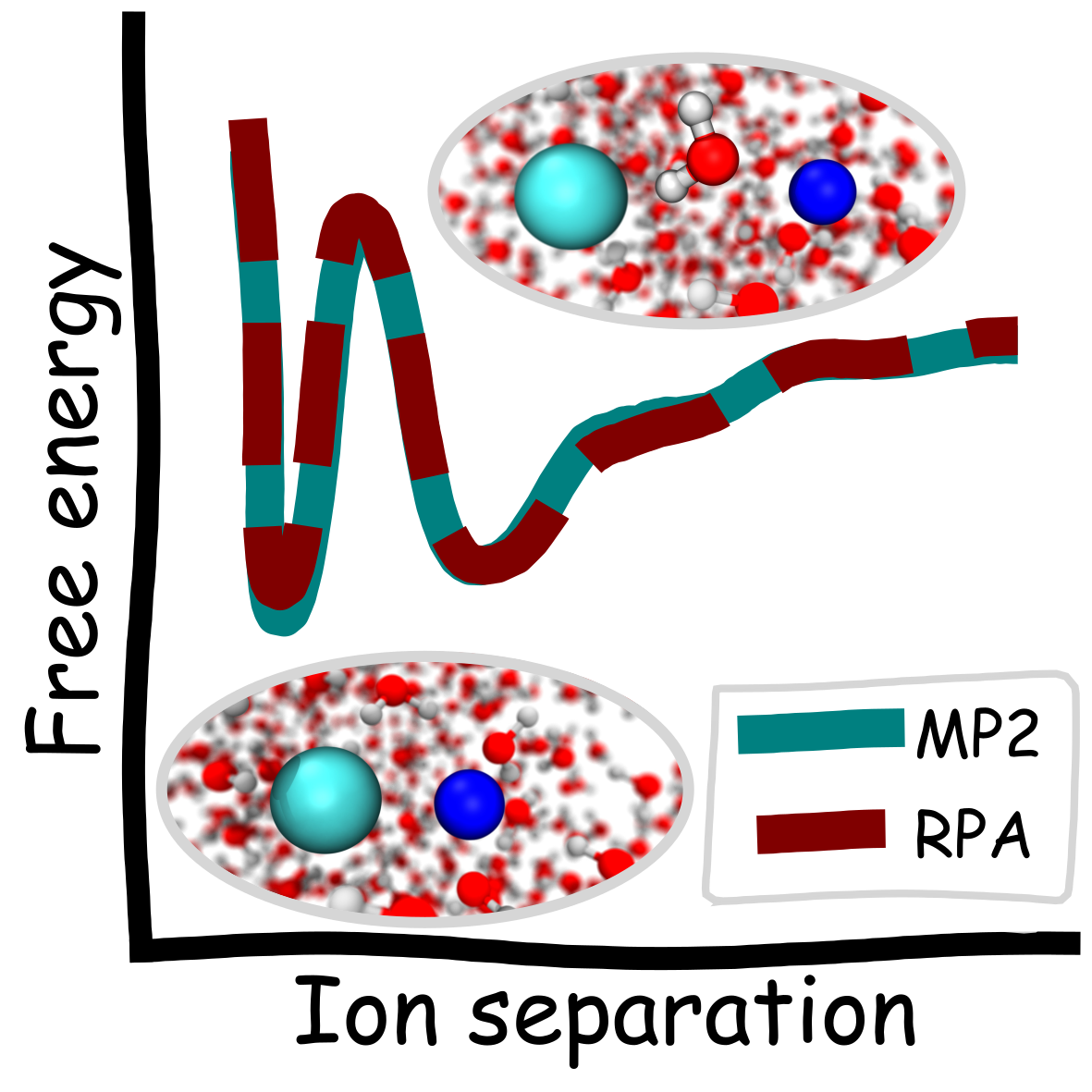
Niamh O’Neill, Benjamin X. Shi, Kara D. Fong, Angelos Michaelides, Christoph Schran
To Pair or not to Pair? Machine-Learned Explicitly-Correlated Electronic Structure for NaCl in Water Journal Article
In: The Journal of Physical Chemistry Letters, vol. 15, no. 23, pp. 6081–6091, 2024, (PMID: 38820256).
Abstract | Links | BibTeX | Tags: Ions in Water, Machine Learning Potentials
@article{ONeill/10.1021/acs.jpclett.4c01030,
title = {To Pair or not to Pair? Machine-Learned Explicitly-Correlated Electronic Structure for NaCl in Water},
author = {Niamh O’Neill and Benjamin X. Shi and Kara D. Fong and Angelos Michaelides and Christoph Schran},
url = {https://doi.org/10.1021/acs.jpclett.4c01030},
doi = {10.1021/acs.jpclett.4c01030},
year = {2024},
date = {2024-05-31},
urldate = {2024-05-31},
journal = {The Journal of Physical Chemistry Letters},
volume = {15},
number = {23},
pages = {6081–6091},
abstract = {The extent of ion pairing in solution is an important phenomenon to rationalize transport and thermodynamic properties of electrolytes. A fundamental measure of this pairing is the potential of mean force (PMF) between solvated ions. The relative stabilities of the paired and solvent shared states in the PMF and the barrier between them are highly sensitive to the underlying potential energy surface. However, direct application of accurate electronic structure methods is challenging, since long simulations are required. We develop wave function based machine learning potentials with the random phase approximation (RPA) and second order Møller–Plesset (MP2) perturbation theory for the prototypical system of Na and Cl ions in water. We show both methods in agreement, predicting the paired and solvent shared states to have similar energies (within 0.2 kcal/mol). We also provide the same benchmarks for different DFT functionals as well as insight into the PMF based on simple analyses of the interactions in the system.},
note = {PMID: 38820256},
keywords = {Ions in Water, Machine Learning Potentials},
pubstate = {published},
tppubtype = {article}
}
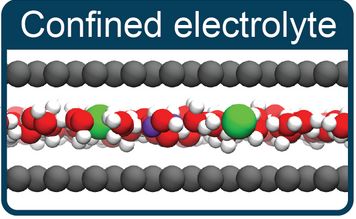
Kara D. Fong, Barbara Sumić, Niamh O’Neill, Christoph Schran, Clare P. Grey, Angelos Michaelides
The Interplay of Solvation and Polarization Effects on Ion Pairing in Nanoconfined Electrolytes Journal Article
In: Nano Letters, vol. 24, no. 16, pp. 5024–5030, 2024, (PMID: 38592099).
Abstract | Links | BibTeX | Tags: Confinement, Hydrogen bonding, Ions in Water, Machine Learning Potentials
@article{Fong/10.1021/acs.nanolett.4c00890,
title = {The Interplay of Solvation and Polarization Effects on Ion Pairing in Nanoconfined Electrolytes},
author = {Kara D. Fong and Barbara Sumić and Niamh O’Neill and Christoph Schran and Clare P. Grey and Angelos Michaelides},
url = {https://doi.org/10.1021/acs.nanolett.4c00890},
doi = {10.1021/acs.nanolett.4c00890},
year = {2024},
date = {2024-04-09},
urldate = {2024-04-09},
journal = {Nano Letters},
volume = {24},
number = {16},
pages = {5024–5030},
abstract = {The nature of ion–ion interactions in electrolytes confined to nanoscale pores has important implications for energy storage and separation technologies. However, the physical effects dictating the structure of nanoconfined electrolytes remain debated. Here we employ machine-learning-based molecular dynamics simulations to investigate ion–ion interactions with density functional theory level accuracy in a prototypical confined electrolyte, aqueous NaCl within graphene slit pores. We find that the free energy of ion pairing in highly confined electrolytes deviates substantially from that in bulk solutions, observing a decrease in contact ion pairing but an increase in solvent-separated ion pairing. These changes arise from an interplay of ion solvation effects and graphene’s electronic structure. Notably, the behavior observed from our first-principles-level simulations is not reproduced even qualitatively with the classical force fields conventionally used to model these systems. The insight provided in this work opens new avenues for predicting and controlling the structure of nanoconfined electrolytes.},
note = {PMID: 38592099},
keywords = {Confinement, Hydrogen bonding, Ions in Water, Machine Learning Potentials},
pubstate = {published},
tppubtype = {article}
}
2021
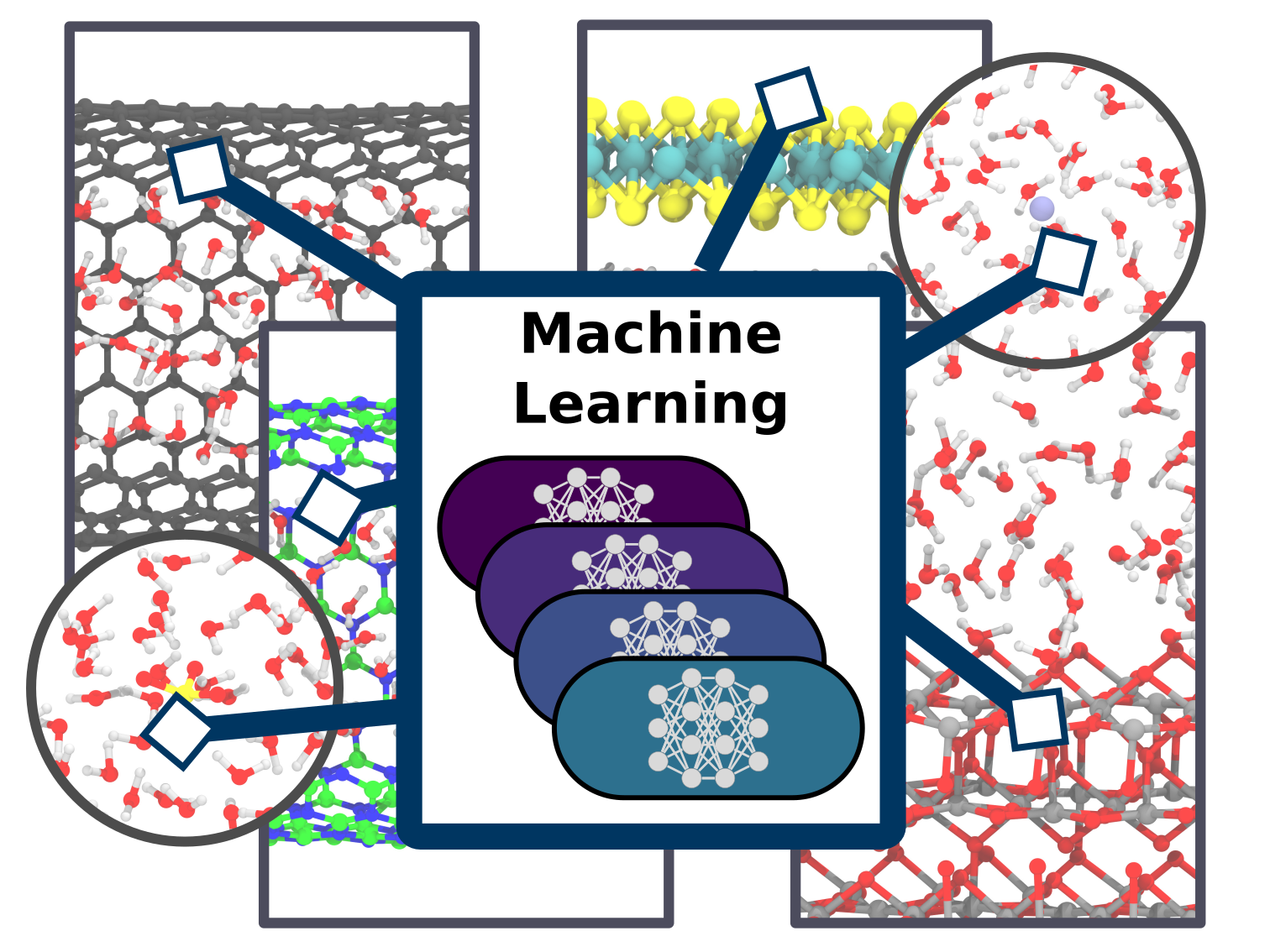
Christoph Schran, Fabian L. Thiemann, Patrick Rowe, Erich A. Müller, Ondrej Marsalek, Angelos Michaelides
Machine learning potentials for complex aqueous systems made simple Journal Article
In: Proc. Natl. Acad. Sci., vol. 118, no. 38, pp. e2110077118, 2021, ISSN: 0027-8424.
Abstract | Links | BibTeX | Tags: Confinement, Ions in Water, Machine Learning Potentials, Water, Water at Interfaces
@article{Schran2021/10.1073/PNAS.2110077118,
title = {Machine learning potentials for complex aqueous systems made simple},
author = {Christoph Schran and Fabian L. Thiemann and Patrick Rowe and Erich A. Müller and Ondrej Marsalek and Angelos Michaelides},
doi = {10.1073/PNAS.2110077118},
issn = {0027-8424},
year = {2021},
date = {2021-09-01},
urldate = {2021-09-01},
journal = {Proc. Natl. Acad. Sci.},
volume = {118},
number = {38},
pages = {e2110077118},
publisher = {National Academy of Sciences},
abstract = {Simulation techniques based on accurate and efficient representations of potential energy surfaces are urgently needed for the understanding of complex aqueous systems such as solid-liquid interfaces. Here, we present a machine learning framework that enables the efficient development and validation of models for complex aqueous systems. Instead of trying to deliver a globally-optimal machine learning potential, we propose to develop models applicable to specific thermodynamic state points in a simple and user-friendly process. After an initial ab initio simulation, a machine learning potential is constructed with minimum human effort through a data-driven active learning protocol. Such models can afterwards be applied in exhaustive simulations to provide reliable answers for the scientific question at hand. We showcase this methodology on a diverse set of aqueous systems with increasing degrees of complexity. The systems chosen here comprise water with different ions in solution, water on a titanium dioxide surface, as well as water confined in nanotubes and between molybdenum disulfide sheets. Highlighting the accuracy of our approach compared to the ab initio reference, the resulting models are evaluated in detail with an automated validation protocol that includes structural and dynamical properties and the precision of the force prediction of the models. Finally, we demonstrate the capabilities of our approach for the description of water on the rutile titanium dioxide (110) surface to analyze structure and mobility of water on this surface. Such machine learning models provide a straightforward and uncomplicated but accurate extension of simulation time and length scales for complex systems.},
keywords = {Confinement, Ions in Water, Machine Learning Potentials, Water, Water at Interfaces},
pubstate = {published},
tppubtype = {article}
}
2017
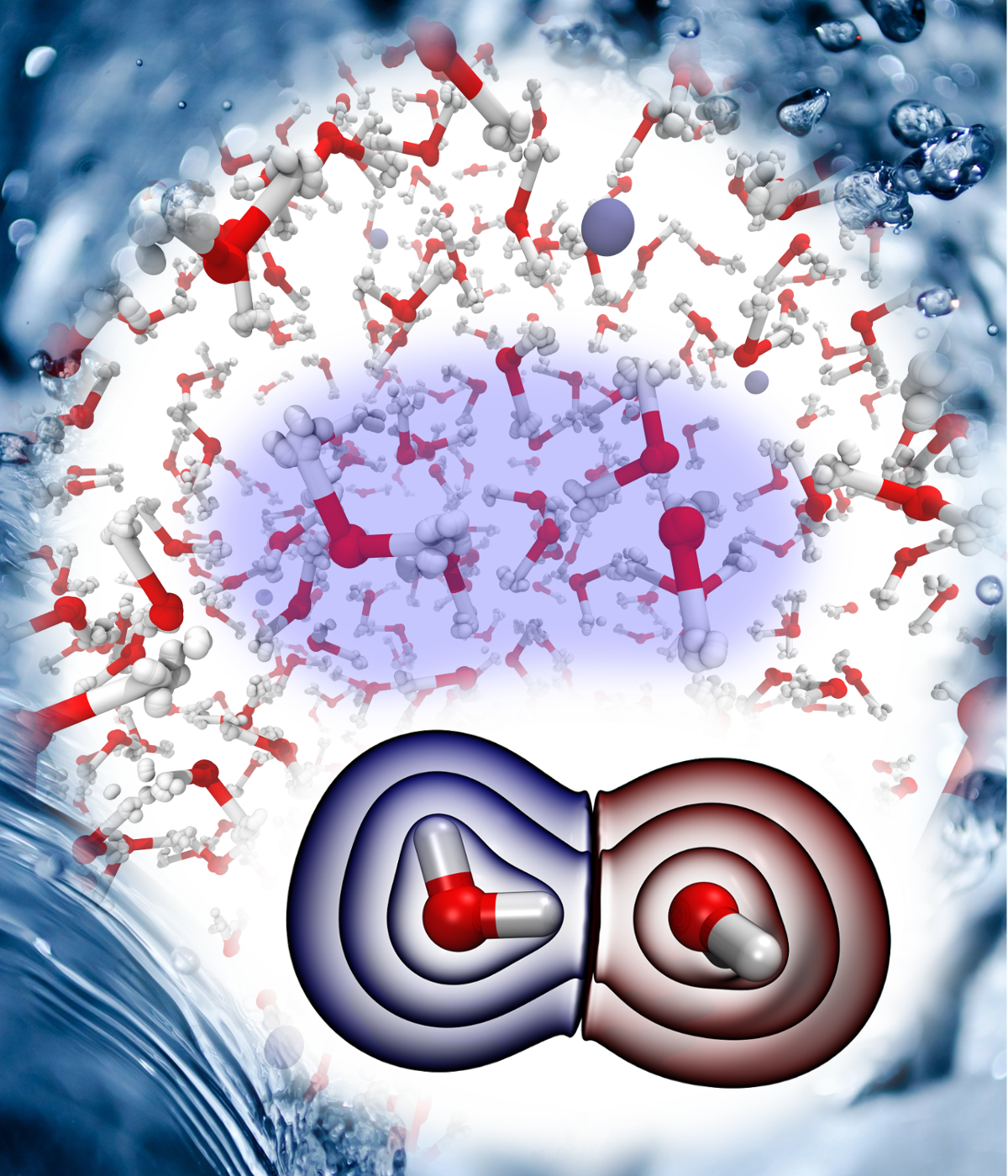
Christoph Schran, Ondrej Marsalek, Thomas E. Markland
Unravelling the influence of quantum proton delocalization on electronic charge transfer through the hydrogen bond Journal Article
In: Chem. Phys. Lett., vol. 678, pp. 289–295, 2017, ISSN: 00092614.
Abstract | Links | BibTeX | Tags: Charge transfer, Hydrogen bonding, Ions in Water, Nuclear quantum effects, Water
@article{Schran2017/10.1016/j.cplett.2017.04.034,
title = {Unravelling the influence of quantum proton delocalization on electronic charge transfer through the hydrogen bond},
author = {Christoph Schran and Ondrej Marsalek and Thomas E. Markland},
doi = {10.1016/j.cplett.2017.04.034},
issn = {00092614},
year = {2017},
date = {2017-06-01},
urldate = {2017-06-01},
journal = {Chem. Phys. Lett.},
volume = {678},
pages = {289–295},
abstract = {Upon hydrogen bond formation, electronic charge density is transferred between the donor and acceptor, impacting processes ranging from hydration to spectroscopy. Here we use ab initio path integral simulations to elucidate the role of nuclear quantum effects in determining the charge transfer in a range of hydrogen bonded species in the gas and liquid phase. We show that the quantization of the nuclei gives rise to large changes in the magnitude of the charge transfer as well as its temperature dependence. We then explain how a single geometric parameter determines the charge transfer through the hydrogen bond. These results thus demonstrate that nuclear quantum effects are vital for the accurate description of charge transfer and offer a physically transparent way to understand how hydrogen bonding gives rise to it.},
keywords = {Charge transfer, Hydrogen bonding, Ions in Water, Nuclear quantum effects, Water},
pubstate = {published},
tppubtype = {article}
}