2024
Samuel G. H. Brookes, Venkat Kapil, Christoph Schran, Angelos Michaelides
The wetting of H$_2$O by CO$_2$ Journal Article
In: J. Chem. Phys., vol. 161, no. 8, pp. 084711, 2024, ISSN: 10897690.
Abstract | Links | BibTeX | Tags: Machine Learning Potentials, Water, Water at Interfaces
@article{Brookes2024/10.1063/5.0224230,
title = {The wetting of H$_2$O by CO$_2$},
author = {Samuel G. H. Brookes and Venkat Kapil and Christoph Schran and Angelos Michaelides},
url = {/aip/jcp/article/161/8/084711/3309975/The-wetting-of-H2O-by-CO2},
doi = {10.1063/5.0224230},
issn = {10897690},
year = {2024},
date = {2024-08-28},
urldate = {2024-08-28},
journal = {J. Chem. Phys.},
volume = {161},
number = {8},
pages = {084711},
publisher = {American Institute of Physics},
abstract = {Biphasic interfaces are complex but fascinating regimes that display a number of properties distinct from those of the bulk. The CO2-H2O interface, in particular, has been the subject of a number of studies on account of its importance for the carbon life cycle as well as carbon capture and sequestration schemes. Despite this attention, there remain a number of open questions on the nature of the CO2-H2O interface, particularly concerning the interfacial tension and phase behavior of CO2 at the interface. In this paper, we seek to address these ambiguities using ab initio-quality simulations. Harnessing the benefits of machine-learned potentials and enhanced statistical sampling methods, we present an ab initio-level description of the CO2-H2O interface. Interfacial tensions are predicted from 1 to 500 bars and found to be in close agreement with experiment at pressures for which experimental data are available. Structural analyses indicate the buildup of an adsorbed, saturated CO2 film forming at a low pressure (20 bars) with properties similar to those of the bulk liquid, but preferential perpendicular alignment with respect to the interface. The CO2 monolayer buildup coincides with a reduced structuring of water molecules close to the interface. This study highlights the predictive nature of machine-learned potentials for complex macroscopic properties of biphasic interfaces, and the mechanistic insight obtained into carbon dioxide aggregation at the water interface is of high relevance for geoscience, climate research, and materials science.},
keywords = {Machine Learning Potentials, Water, Water at Interfaces},
pubstate = {published},
tppubtype = {article}
}
2022
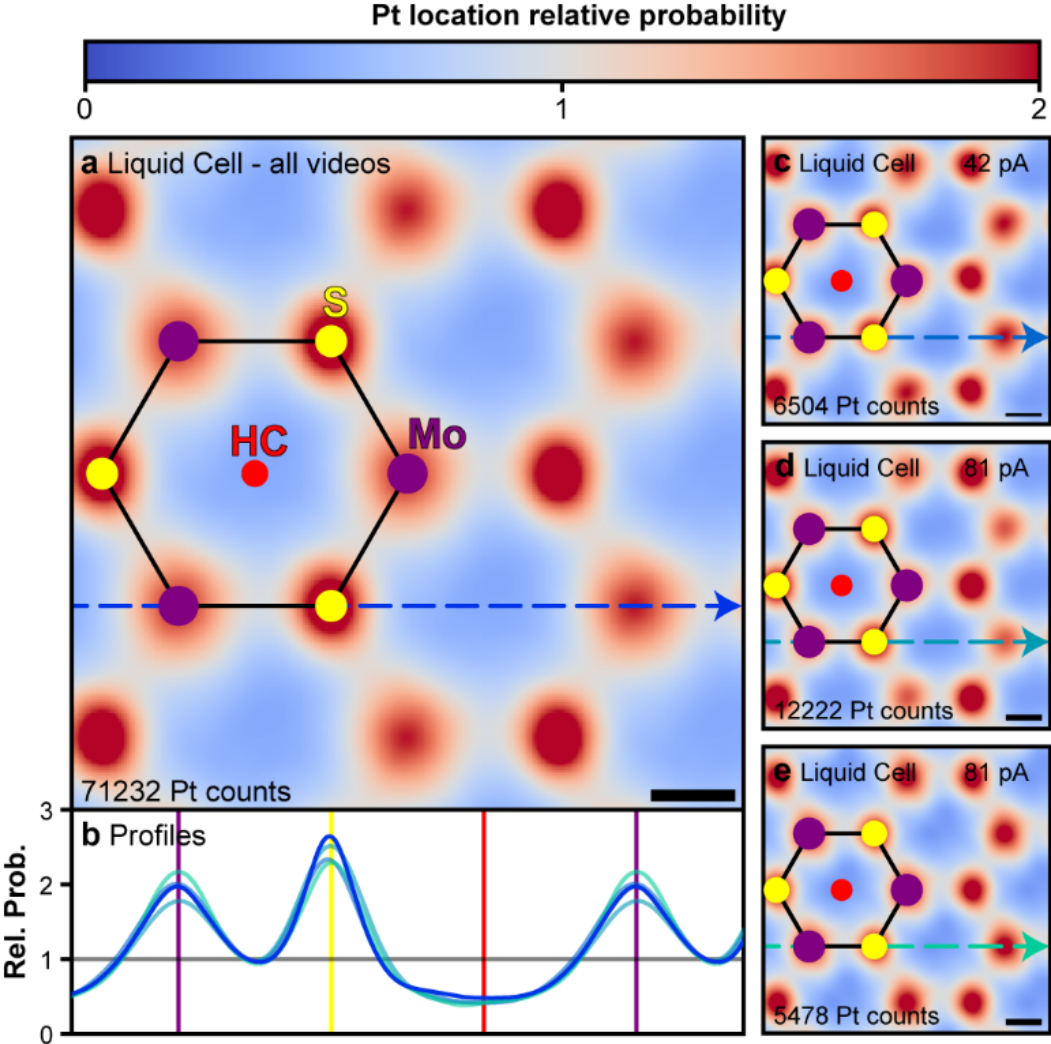
Nick Clark, Daniel J. Kelly, Mingwei Zhou, Yi-Chao Zou, Chang Woo Myung, David G. Hopkinson, Christoph Schran, Angelos Michaelides, Roman Gorbachev, Sarah J. Haigh
Tracking single adatoms in liquid in a Transmission Electron Microscope Journal Article
In: Nature, pp. 1–3, 2022, ISSN: 1476-4687.
Abstract | Links | BibTeX | Tags: Two-dimensional materials, Water at Interfaces
@article{Clark2022/10.1038/s41586-022-05130-0,
title = {Tracking single adatoms in liquid in a Transmission Electron Microscope},
author = {Nick Clark and Daniel J. Kelly and Mingwei Zhou and Yi-Chao Zou and Chang Woo Myung and David G. Hopkinson and Christoph Schran and Angelos Michaelides and Roman Gorbachev and Sarah J. Haigh},
doi = {10.1038/s41586-022-05130-0},
issn = {1476-4687},
year = {2022},
date = {2022-07-01},
urldate = {2022-07-01},
journal = {Nature},
pages = {1–3},
publisher = {Nature Publishing Group},
abstract = {Single atoms or ions on surfaces affect processes from nucleation1 to electrochemical reactions2 and heterogeneous catalysis3. Transmission electron microscopy (TEM) is a leading approach for visualizing single atoms on a variety of substrates4,5. It conventionally requires high vacuum conditions, but has been developed for in situ imaging in liquid and gaseous environments6,7 with a combined spatial and temporal resolution that is unmatched by any other method —notwithstanding concerns about electron beam effects on samples. When imaging in liquid using commercial technologies, electron scattering in the windows enclosing the sample and in the liquid generally limits the achievable resolution to a few nanometres6,8,9. Graphene liquid cells, on the other hand, have enabled atomic resolution imaging of metal nanoparticles in liquids10. Here we show that a double graphene liquid cell, comprised of a central molybdenum disulphide monolayer separated by hexagonal boron nitride spacers from the two enclosing graphene windows, makes it possible to monitor with atomic resolution the dynamics of platinum adatoms on the monolayer in an aqueous salt solution. By imaging over 70,000 single adatom adsorption sites, we compare the site preference and dynamic motion of the adatoms in both a fully hydrated and vacuum state. We find a modified adsorption site distribution and higher diffusivities for the adatoms in liquid phase compared to those in vacuum. This approach paves the way for in situ liquid phase imaging of chemical processes with single atom precision.},
keywords = {Two-dimensional materials, Water at Interfaces},
pubstate = {published},
tppubtype = {article}
}
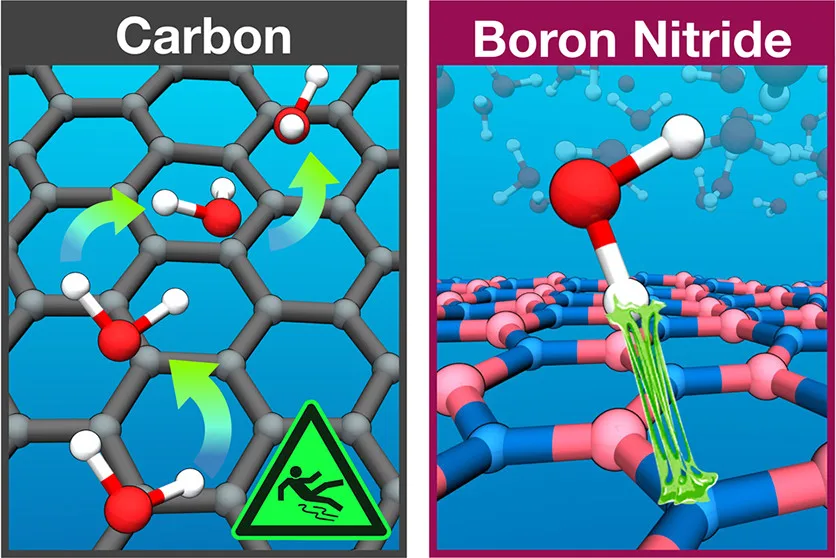
Fabian L. Thiemann, Christoph Schran, Patrick Rowe, Erich A. Müller, Angelos Michaelides
Water flow in single-wall nanotubes: Oxygen makes it slip, hydrogen makes it stick Journal Article
In: ACS Nano, vol. 16, no. 7, pp. 10775–10782, 2022.
Abstract | Links | BibTeX | Tags: Confinement, Hydrogen bonding, Machine Learning Potentials, Water at Interfaces, Water flow
@article{Thiemann2022/10.1021/acsnano.2c02784,
title = {Water flow in single-wall nanotubes: Oxygen makes it slip, hydrogen makes it stick},
author = {Fabian L. Thiemann and Christoph Schran and Patrick Rowe and Erich A. Müller and Angelos Michaelides},
doi = {10.1021/acsnano.2c02784},
year = {2022},
date = {2022-06-01},
urldate = {2022-06-01},
journal = {ACS Nano},
volume = {16},
number = {7},
pages = {10775–10782},
abstract = {Experimental measurements have reported ultra-fast and radius-dependent water transport in carbon nanotubes which are absent in boron nitride nanotubes. Despite considerable effort, the origin of this contrasting (and fascinating) behaviour is not understood. Here, with the aid of machine learning-based molecular dynamics simulations that deliver first-principles accuracy, we investigate water transport in single-wall carbon and boron nitride nanotubes. Our simulations reveal a large, radius-dependent hydrodynamic slippage on both materials with water experiencing indeed a $backslashapprox 5$ times lower friction on carbon surfaces compared to boron nitride. Analysis of the diffusion mechanisms across the two materials reveals that the fast water transport on carbon is governed by facile oxygen motion, whereas the higher friction on boron nitride arises from specific hydrogen-nitrogen interactions. This work not only delivers a clear reference of unprecedented accuracy for water flow in single-wall nanotubes, but also provides detailed mechanistic insight into its radius and material dependence for future technological application.},
keywords = {Confinement, Hydrogen bonding, Machine Learning Potentials, Water at Interfaces, Water flow},
pubstate = {published},
tppubtype = {article}
}
2021
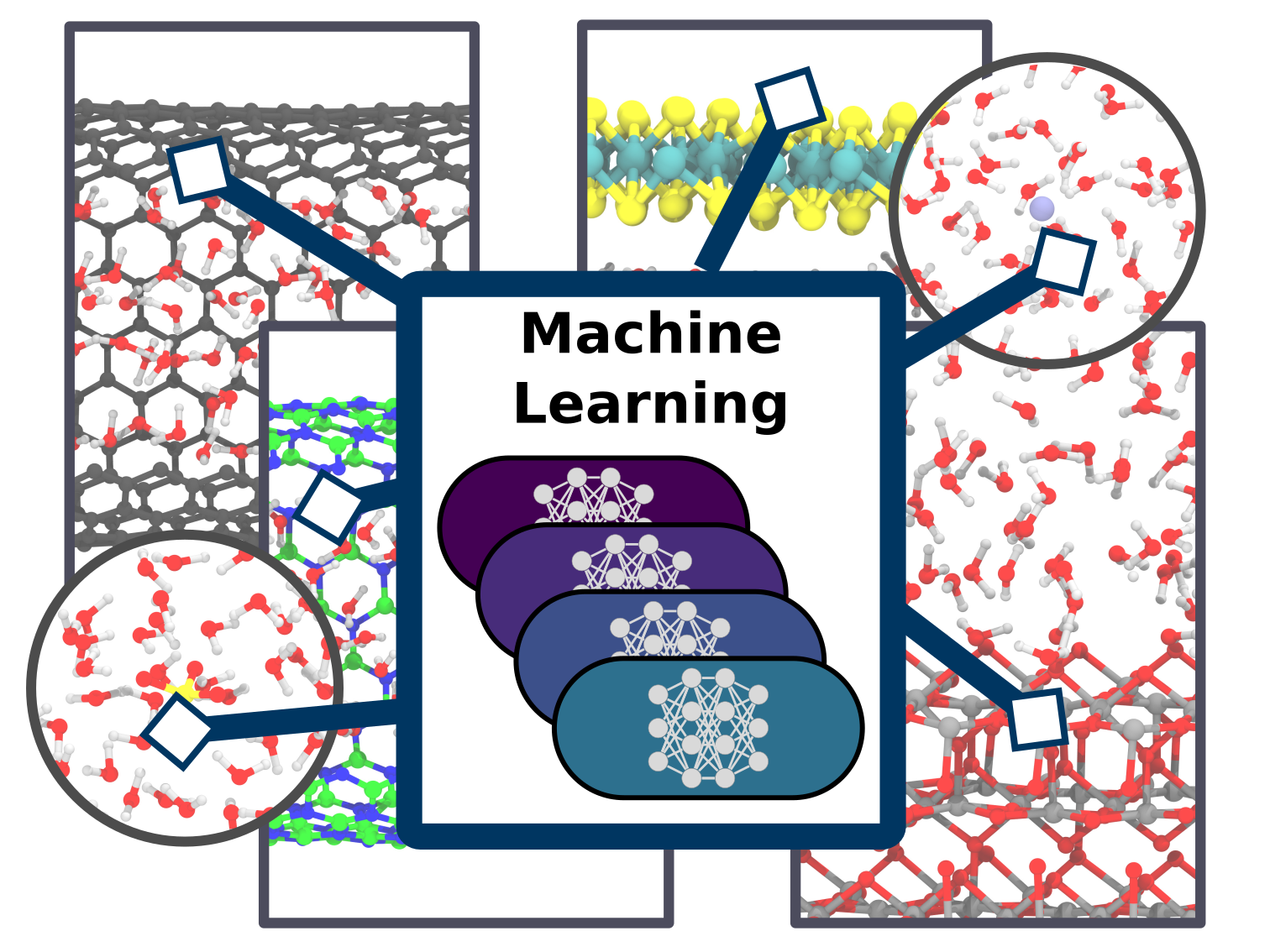
Christoph Schran, Fabian L. Thiemann, Patrick Rowe, Erich A. Müller, Ondrej Marsalek, Angelos Michaelides
Machine learning potentials for complex aqueous systems made simple Journal Article
In: Proc. Natl. Acad. Sci., vol. 118, no. 38, pp. e2110077118, 2021, ISSN: 0027-8424.
Abstract | Links | BibTeX | Tags: Confinement, Ions in Water, Machine Learning Potentials, Water, Water at Interfaces
@article{Schran2021/10.1073/PNAS.2110077118,
title = {Machine learning potentials for complex aqueous systems made simple},
author = {Christoph Schran and Fabian L. Thiemann and Patrick Rowe and Erich A. Müller and Ondrej Marsalek and Angelos Michaelides},
doi = {10.1073/PNAS.2110077118},
issn = {0027-8424},
year = {2021},
date = {2021-09-01},
urldate = {2021-09-01},
journal = {Proc. Natl. Acad. Sci.},
volume = {118},
number = {38},
pages = {e2110077118},
publisher = {National Academy of Sciences},
abstract = {Simulation techniques based on accurate and efficient representations of potential energy surfaces are urgently needed for the understanding of complex aqueous systems such as solid-liquid interfaces. Here, we present a machine learning framework that enables the efficient development and validation of models for complex aqueous systems. Instead of trying to deliver a globally-optimal machine learning potential, we propose to develop models applicable to specific thermodynamic state points in a simple and user-friendly process. After an initial ab initio simulation, a machine learning potential is constructed with minimum human effort through a data-driven active learning protocol. Such models can afterwards be applied in exhaustive simulations to provide reliable answers for the scientific question at hand. We showcase this methodology on a diverse set of aqueous systems with increasing degrees of complexity. The systems chosen here comprise water with different ions in solution, water on a titanium dioxide surface, as well as water confined in nanotubes and between molybdenum disulfide sheets. Highlighting the accuracy of our approach compared to the ab initio reference, the resulting models are evaluated in detail with an automated validation protocol that includes structural and dynamical properties and the precision of the force prediction of the models. Finally, we demonstrate the capabilities of our approach for the description of water on the rutile titanium dioxide (110) surface to analyze structure and mobility of water on this surface. Such machine learning models provide a straightforward and uncomplicated but accurate extension of simulation time and length scales for complex systems.},
keywords = {Confinement, Ions in Water, Machine Learning Potentials, Water, Water at Interfaces},
pubstate = {published},
tppubtype = {article}
}