2024
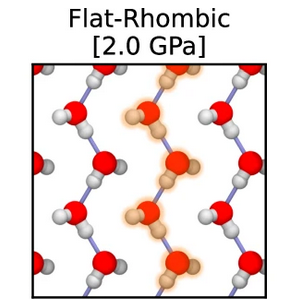
Pavan Ravindra, Xavier R. Advincula, Christoph Schran, Angelos Michaelides, Venkat Kapil
Quasi-one-dimensional hydrogen bonding in nanoconfined ice Journal Article
In: Nat. Commun., vol. 15, no. 1, pp. 1–9, 2024, ISSN: 20411723.
Abstract | Links | BibTeX | Tags: Confinement, Hydrogen bonding, Machine Learning Potentials, Water
@article{Ravindra2024/10.1038/s41467-024-51124-z,
title = {Quasi-one-dimensional hydrogen bonding in nanoconfined ice},
author = {Pavan Ravindra and Xavier R. Advincula and Christoph Schran and Angelos Michaelides and Venkat Kapil},
url = {https://www.nature.com/articles/s41467-024-51124-z},
doi = {10.1038/s41467-024-51124-z},
issn = {20411723},
year = {2024},
date = {2024-08-24},
urldate = {2024-08-24},
journal = {Nat. Commun.},
volume = {15},
number = {1},
pages = {1–9},
publisher = {Nature Publishing Group},
abstract = {The Bernal-Fowler ice rules stipulate that each water molecule in an ice crystal should form four hydrogen bonds. However, in extreme or constrained conditions, the arrangement of water molecules deviates from conventional ice rules, resulting in properties significantly different from bulk water. In this study, we employ machine learning-driven first-principles simulations to identify a new stabilization mechanism in nanoconfined ice phases. Instead of forming four hydrogen bonds, nanoconfined crystalline ice can form a quasi-one-dimensional hydrogen-bonded structure that exhibits only two hydrogen bonds per water molecule. These structures consist of strongly hydrogen-bonded linear chains of water molecules that zig-zag along one dimension, stabilized by van der Waals interactions that stack these chains along the other dimension. The unusual interplay of hydrogen bonding and van der Waals interactions in nanoconfined ice results in atypical proton behavior such as potential ferroelectric behavior, low dielectric response, and long-range proton dynamics.},
keywords = {Confinement, Hydrogen bonding, Machine Learning Potentials, Water},
pubstate = {published},
tppubtype = {article}
}
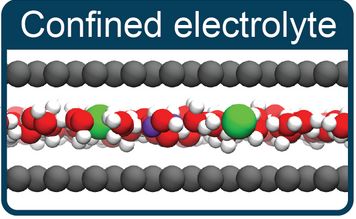
Kara D. Fong, Barbara Sumić, Niamh O’Neill, Christoph Schran, Clare P. Grey, Angelos Michaelides
The Interplay of Solvation and Polarization Effects on Ion Pairing in Nanoconfined Electrolytes Journal Article
In: Nano Letters, vol. 24, no. 16, pp. 5024–5030, 2024, (PMID: 38592099).
Abstract | Links | BibTeX | Tags: Confinement, Hydrogen bonding, Ions in Water, Machine Learning Potentials
@article{Fong/10.1021/acs.nanolett.4c00890,
title = {The Interplay of Solvation and Polarization Effects on Ion Pairing in Nanoconfined Electrolytes},
author = {Kara D. Fong and Barbara Sumić and Niamh O’Neill and Christoph Schran and Clare P. Grey and Angelos Michaelides},
url = {https://doi.org/10.1021/acs.nanolett.4c00890},
doi = {10.1021/acs.nanolett.4c00890},
year = {2024},
date = {2024-04-09},
urldate = {2024-04-09},
journal = {Nano Letters},
volume = {24},
number = {16},
pages = {5024–5030},
abstract = {The nature of ion–ion interactions in electrolytes confined to nanoscale pores has important implications for energy storage and separation technologies. However, the physical effects dictating the structure of nanoconfined electrolytes remain debated. Here we employ machine-learning-based molecular dynamics simulations to investigate ion–ion interactions with density functional theory level accuracy in a prototypical confined electrolyte, aqueous NaCl within graphene slit pores. We find that the free energy of ion pairing in highly confined electrolytes deviates substantially from that in bulk solutions, observing a decrease in contact ion pairing but an increase in solvent-separated ion pairing. These changes arise from an interplay of ion solvation effects and graphene’s electronic structure. Notably, the behavior observed from our first-principles-level simulations is not reproduced even qualitatively with the classical force fields conventionally used to model these systems. The insight provided in this work opens new avenues for predicting and controlling the structure of nanoconfined electrolytes.},
note = {PMID: 38592099},
keywords = {Confinement, Hydrogen bonding, Ions in Water, Machine Learning Potentials},
pubstate = {published},
tppubtype = {article}
}
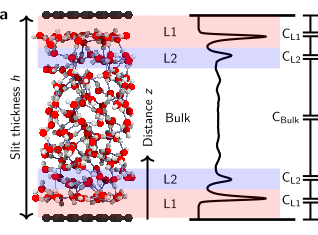
Thomas Dufils, Christoph Schran, Ji Chen, Andre K. Geim, Laura Fumagalli, Angelos Michaelides
Origin of dielectric polarization suppression in confined water from first principles Journal Article
In: Chem. Sci., vol. 15, iss. 2, pp. 516–527, 2024.
Abstract | Links | BibTeX | Tags: AIMD, Confinement, Hydrogen bonding, Water
@article{Dufils2024/10.1039/D3SC04740G,
title = {Origin of dielectric polarization suppression in confined water from first principles},
author = {Thomas Dufils and Christoph Schran and Ji Chen and Andre K. Geim and Laura Fumagalli and Angelos Michaelides},
url = {http://dx.doi.org/10.1039/D3SC04740G},
doi = {10.1039/D3SC04740G},
year = {2024},
date = {2024-01-01},
urldate = {2024-01-01},
journal = {Chem. Sci.},
volume = {15},
issue = {2},
pages = {516–527},
publisher = {The Royal Society of Chemistry},
abstract = {It has long been known that the dielectric constant of confined water should be different from that in bulk. Recent experiments have shown that it is vanishingly small, however the origin of the phenomenon remains unclear. Here we used ab initio molecular dynamics simulations (AIMD) and AIMD-trained machine-learning potentials to understand water's structure and electronic properties underpinning this effect. For the graphene and hexagonal boron-nitride substrates considered, we find that it originates in the spontaneous anti-parallel alignment of the water dipoles in the first two water layers near the solid interface. The interfacial layers exhibit net ferroelectric ordering, resulting in an overall anti-ferroelectric arrangement of confined water. Together with constrained hydrogen-bonding orientations, this leads to much reduced out-of-plane polarization. Furthermore, we directly contrast AIMD and simple classical force-field simulations, revealing important differences. This work offers insight into a property of water that is critical in modulating surface forces, the electric-double-layer formation and molecular solvation, and shows a way to compute it.},
keywords = {AIMD, Confinement, Hydrogen bonding, Water},
pubstate = {published},
tppubtype = {article}
}
2022
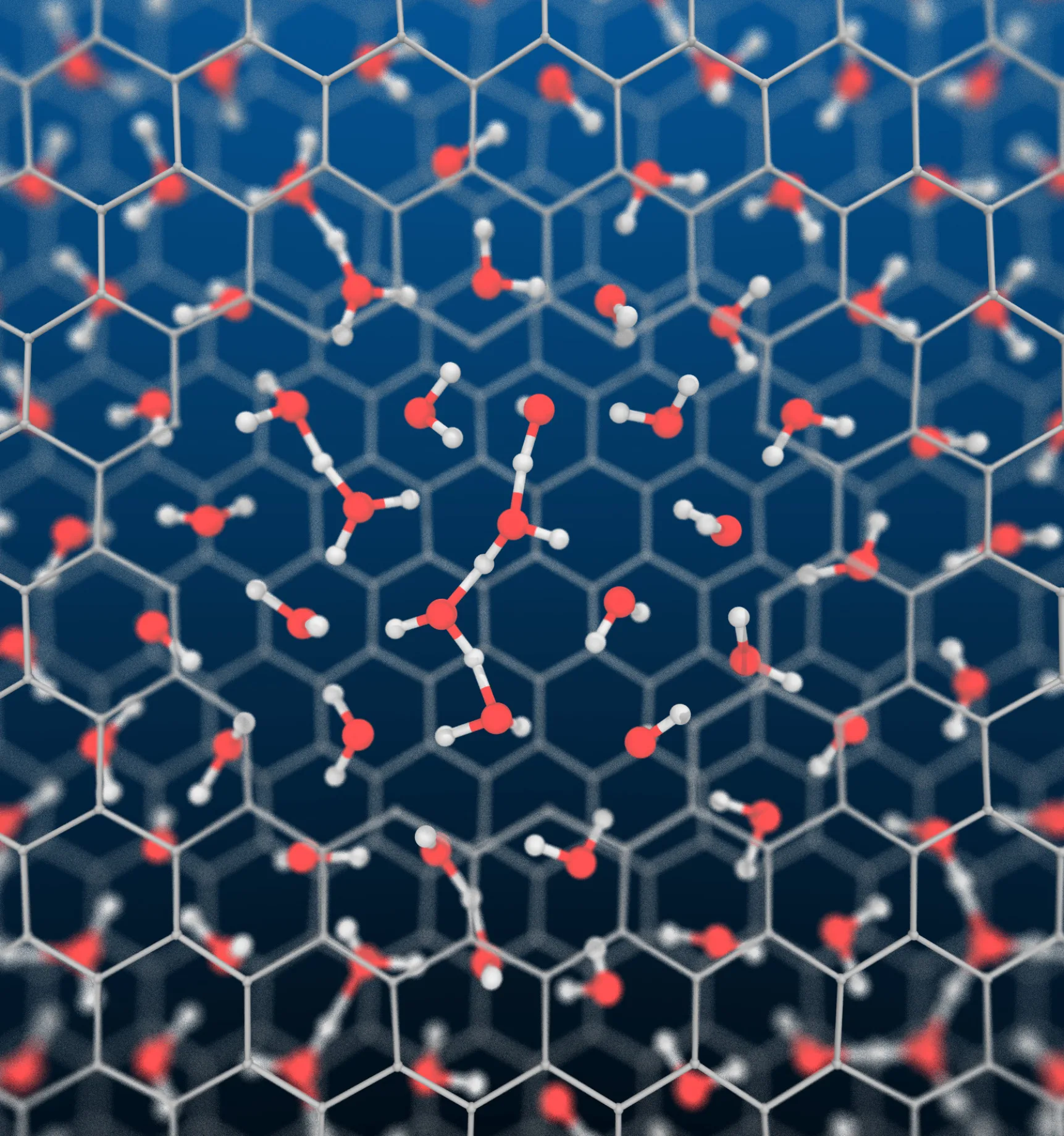
Venkat Kapil, Christoph Schran, Andrea Zen, Ji Chen, Chris J. Pickard, Angelos Michaelides
The first-principles phase diagram of monolayer nanoconfined water Journal Article
In: Nature, vol. 609, pp. 512-516, 2022, ISSN: 1476-4687.
Abstract | Links | BibTeX | Tags: Confinement, Machine Learning Potentials, Water
@article{Kapil2022/10.1038/S41586-022-05036-X,
title = {The first-principles phase diagram of monolayer nanoconfined water},
author = {Venkat Kapil and Christoph Schran and Andrea Zen and Ji Chen and Chris J. Pickard and Angelos Michaelides},
doi = {10.1038/S41586-022-05036-X},
issn = {1476-4687},
year = {2022},
date = {2022-09-01},
urldate = {2022-09-01},
journal = {Nature},
volume = {609},
pages = {512-516},
publisher = {Nature Publishing Group},
abstract = {Water in nanoscale cavities is ubiquitous and of central importance to everyday phenomena in geology and biology. However, the properties of nanoscale water can be substantially different from those of bulk water, as shown, for example, by the anomalously low dielectric constant of water in nanochannels1, near frictionless water flow2 or the possible existence of a square ice phase3. Such properties suggest that nanoconfined water could be engineered for technological applications in nanofluidics4, electrolyte materials5 and water desalination6. Unfortunately, challenges in experimentally characterizing water at the nanoscale and the high cost of first-principles simulations have prevented the molecular-level understanding required to control the behaviour of water. Here we combine a range of computational approaches to enable a first-principles-level investigation of a single layer of water within a graphene-like channel. We find that monolayer water exhibits surprisingly rich and diverse phase behaviour that is highly sensitive to temperature and the van der Waals pressure acting within the nanochannel. In addition to multiple molecular phases with melting temperatures varying non-monotonically by more than 400 kelvins with pressure, we predict a hexatic phase, which is an intermediate between a solid and a liquid, and a superionic phase with a high electrical conductivity exceeding that of battery materials. Notably, this suggests that nanoconfinement could be a promising route towards superionic behaviour under easily accessible conditions. Monolayer water exhibits rich and diverse phase behaviour that is highly sensitive to temperature and the van der Waals pressure acting within the nanochannel.},
keywords = {Confinement, Machine Learning Potentials, Water},
pubstate = {published},
tppubtype = {article}
}
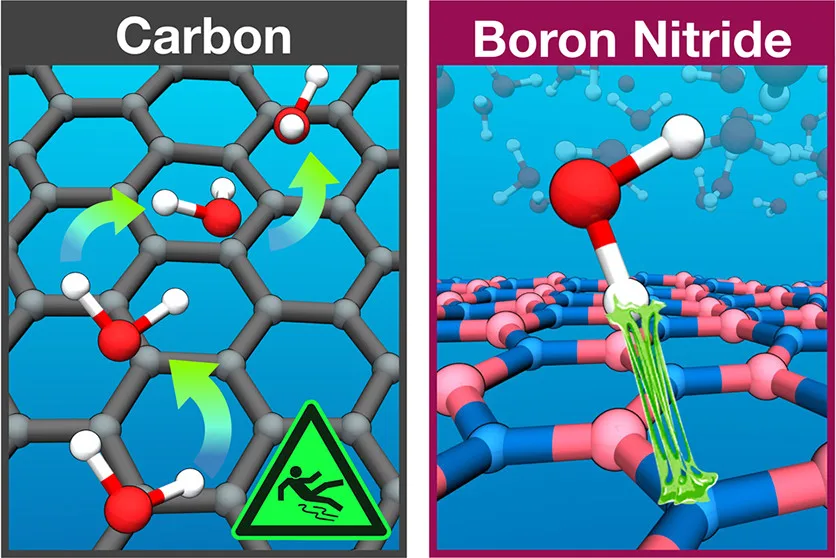
Fabian L. Thiemann, Christoph Schran, Patrick Rowe, Erich A. Müller, Angelos Michaelides
Water flow in single-wall nanotubes: Oxygen makes it slip, hydrogen makes it stick Journal Article
In: ACS Nano, vol. 16, no. 7, pp. 10775–10782, 2022.
Abstract | Links | BibTeX | Tags: Confinement, Hydrogen bonding, Machine Learning Potentials, Water at Interfaces, Water flow
@article{Thiemann2022/10.1021/acsnano.2c02784,
title = {Water flow in single-wall nanotubes: Oxygen makes it slip, hydrogen makes it stick},
author = {Fabian L. Thiemann and Christoph Schran and Patrick Rowe and Erich A. Müller and Angelos Michaelides},
doi = {10.1021/acsnano.2c02784},
year = {2022},
date = {2022-06-01},
urldate = {2022-06-01},
journal = {ACS Nano},
volume = {16},
number = {7},
pages = {10775–10782},
abstract = {Experimental measurements have reported ultra-fast and radius-dependent water transport in carbon nanotubes which are absent in boron nitride nanotubes. Despite considerable effort, the origin of this contrasting (and fascinating) behaviour is not understood. Here, with the aid of machine learning-based molecular dynamics simulations that deliver first-principles accuracy, we investigate water transport in single-wall carbon and boron nitride nanotubes. Our simulations reveal a large, radius-dependent hydrodynamic slippage on both materials with water experiencing indeed a $backslashapprox 5$ times lower friction on carbon surfaces compared to boron nitride. Analysis of the diffusion mechanisms across the two materials reveals that the fast water transport on carbon is governed by facile oxygen motion, whereas the higher friction on boron nitride arises from specific hydrogen-nitrogen interactions. This work not only delivers a clear reference of unprecedented accuracy for water flow in single-wall nanotubes, but also provides detailed mechanistic insight into its radius and material dependence for future technological application.},
keywords = {Confinement, Hydrogen bonding, Machine Learning Potentials, Water at Interfaces, Water flow},
pubstate = {published},
tppubtype = {article}
}
2021
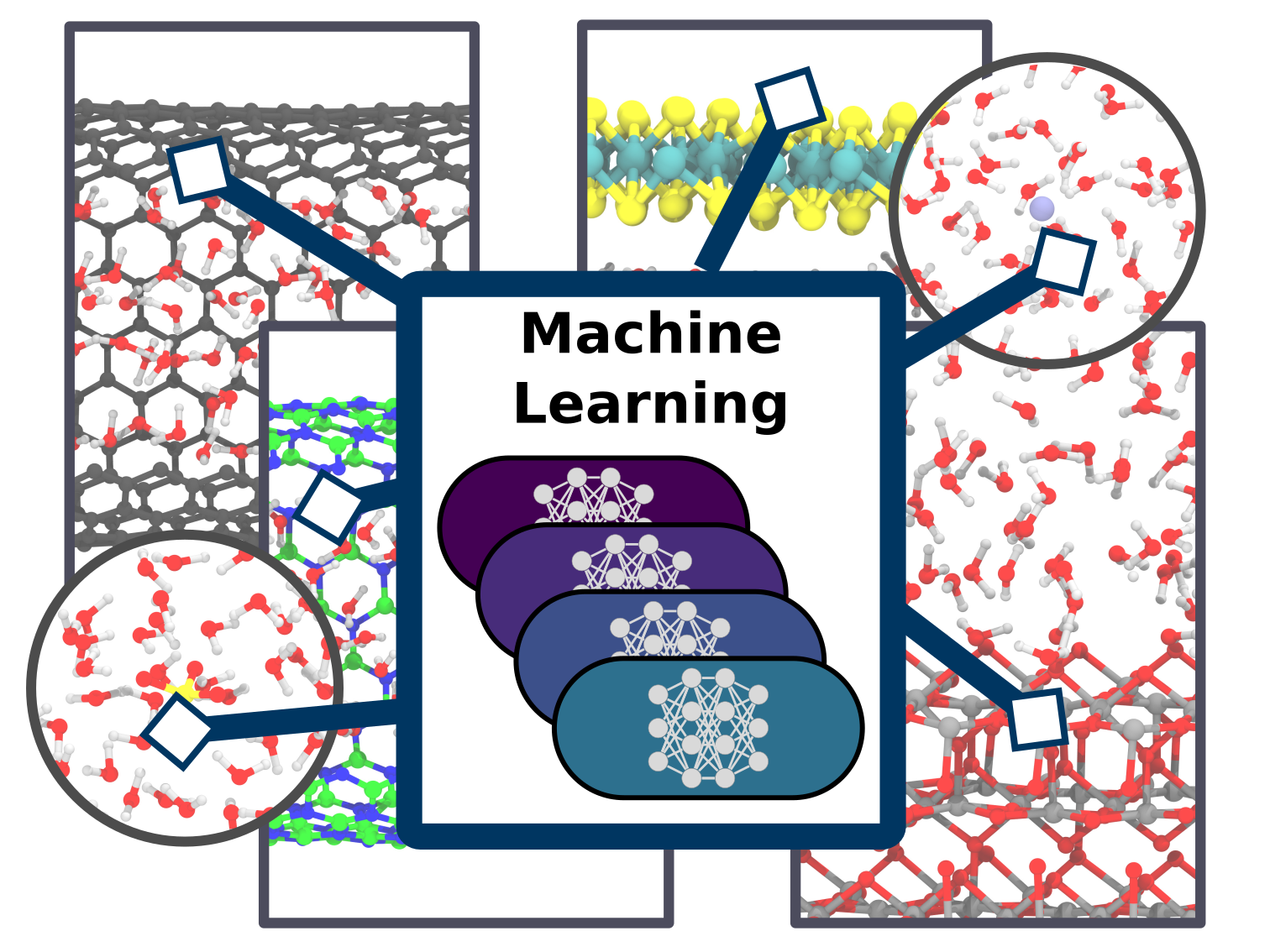
Christoph Schran, Fabian L. Thiemann, Patrick Rowe, Erich A. Müller, Ondrej Marsalek, Angelos Michaelides
Machine learning potentials for complex aqueous systems made simple Journal Article
In: Proc. Natl. Acad. Sci., vol. 118, no. 38, pp. e2110077118, 2021, ISSN: 0027-8424.
Abstract | Links | BibTeX | Tags: Confinement, Ions in Water, Machine Learning Potentials, Water, Water at Interfaces
@article{Schran2021/10.1073/PNAS.2110077118,
title = {Machine learning potentials for complex aqueous systems made simple},
author = {Christoph Schran and Fabian L. Thiemann and Patrick Rowe and Erich A. Müller and Ondrej Marsalek and Angelos Michaelides},
doi = {10.1073/PNAS.2110077118},
issn = {0027-8424},
year = {2021},
date = {2021-09-01},
urldate = {2021-09-01},
journal = {Proc. Natl. Acad. Sci.},
volume = {118},
number = {38},
pages = {e2110077118},
publisher = {National Academy of Sciences},
abstract = {Simulation techniques based on accurate and efficient representations of potential energy surfaces are urgently needed for the understanding of complex aqueous systems such as solid-liquid interfaces. Here, we present a machine learning framework that enables the efficient development and validation of models for complex aqueous systems. Instead of trying to deliver a globally-optimal machine learning potential, we propose to develop models applicable to specific thermodynamic state points in a simple and user-friendly process. After an initial ab initio simulation, a machine learning potential is constructed with minimum human effort through a data-driven active learning protocol. Such models can afterwards be applied in exhaustive simulations to provide reliable answers for the scientific question at hand. We showcase this methodology on a diverse set of aqueous systems with increasing degrees of complexity. The systems chosen here comprise water with different ions in solution, water on a titanium dioxide surface, as well as water confined in nanotubes and between molybdenum disulfide sheets. Highlighting the accuracy of our approach compared to the ab initio reference, the resulting models are evaluated in detail with an automated validation protocol that includes structural and dynamical properties and the precision of the force prediction of the models. Finally, we demonstrate the capabilities of our approach for the description of water on the rutile titanium dioxide (110) surface to analyze structure and mobility of water on this surface. Such machine learning models provide a straightforward and uncomplicated but accurate extension of simulation time and length scales for complex systems.},
keywords = {Confinement, Ions in Water, Machine Learning Potentials, Water, Water at Interfaces},
pubstate = {published},
tppubtype = {article}
}